Big data applications in healthcare industry are revolutionizing the way we approach patient care, operational efficiency, and public health. With the ability to analyze vast amounts of data from diverse sources, healthcare providers can personalize treatment plans and improve outcomes. This transformation is facilitated through real-time monitoring systems, predictive analytics, and innovative research methodologies, which together forge a path toward a more data-driven healthcare environment.
From enhancing personalized medicine to optimizing hospital operations and accelerating drug discovery, big data is at the forefront of a healthcare renaissance. It not only empowers clinicians to make informed decisions but also engages patients in their healthcare journey, ultimately leading to improved satisfaction and health outcomes. The integration of big data into various aspects of healthcare is not just a trend; it’s a fundamental shift in how we understand and manage health.
Big Data in Patient Care
Big data is revolutionizing patient care by facilitating personalized medicine, which tailors treatment to individual patient characteristics. This innovation allows healthcare providers to analyze vast datasets to identify patterns and correlations that can enhance patient outcomes. By leveraging data from various sources, including genetic information, medical histories, and real-time health monitoring, healthcare systems can implement more effective and targeted interventions.Big data enhances personalized medicine in several ways.
Database normalization is an essential process in designing efficient databases. There are several types of database normalization techniques, which help eliminate redundancy and ensure data integrity. By understanding what are the different types of database normalization techniques , developers can create more organized data structures that enhance overall performance.
It enables the integration of diverse data sources, leading to a comprehensive understanding of patient needs and responses. This understanding is pivotal for developing treatment plans that are specifically suited to individual patients rather than adopting a one-size-fits-all approach. For instance, through analyzing genetic data, clinicians can identify which patients are likely to respond positively to specific medications, thereby minimizing adverse effects and improving efficacy.
Real-Time Monitoring Systems Utilizing Big Data
Real-time monitoring systems have emerged as vital tools in patient care, utilizing big data to track patient health metrics continuously. These systems collect data from wearables and connected devices, providing healthcare professionals with immediate insights into a patient’s condition. The importance of these systems lies in their ability to promote proactive care, where potential health issues are identified and addressed before they escalate.Examples of real-time monitoring systems include:
- Wearable Health Devices: Devices like fitness trackers and smartwatches monitor heart rates, physical activity, and even sleep patterns, allowing for immediate feedback and adjustments to care plans.
- Remote Patient Monitoring (RPM): Technologies enabling patients with chronic conditions, such as diabetes or heart disease, to be monitored from home, significantly reducing hospital visits and associated costs.
- Telehealth Platforms: Integrated systems that use big data analytics to provide virtual consultations and continuous patient monitoring, improving access to care while keeping healthcare costs manageable.
Integrating Big Data Analytics into Patient Care Protocols
The integration of big data analytics into patient care protocols is essential for improving health outcomes and operational efficiency. By employing robust data analytics, healthcare providers can refine their treatment methodologies and patient management strategies.Key methods for successful integration include:
- Data-Driven Decision Making: Utilizing analytics to inform clinical decisions, thereby enhancing the quality of care provided to patients.
- Risk Stratification: Analyzing patient data to identify those at high risk for certain conditions, allowing for targeted interventions and preemptive care.
- Care Coordination: Implementing systems that ensure seamless sharing of patient information across various healthcare settings, improving communication among providers and ensuring comprehensive care.
“Big data in healthcare is not just about the amount of data collected, but the actionable insights derived from it that can directly improve patient outcomes.”
Operational Efficiency in Healthcare Facilities
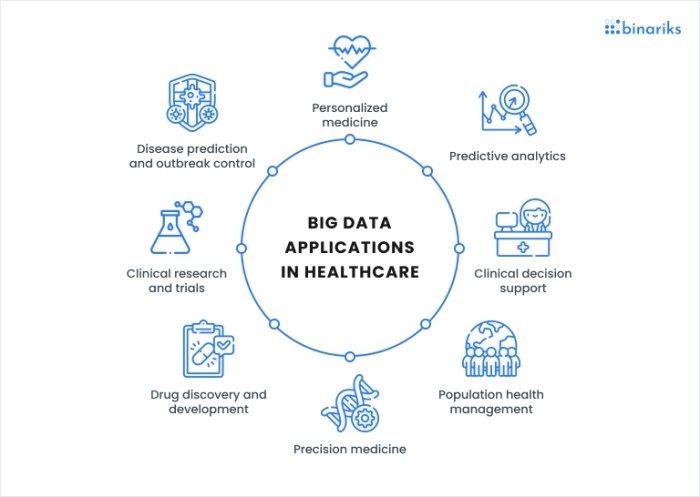
Big data has emerged as a transformative force in the healthcare sector, playing a pivotal role in enhancing operational efficiency within healthcare facilities. By harnessing vast amounts of data, hospitals and clinics can streamline resource management, improve scheduling, and optimize staff allocation, leading to better patient outcomes and cost savings. The integration of predictive analytics further revolutionizes emergency room operations, ensuring prompt and effective care delivery.
Optimizing Hospital Resource Management
Effective resource management is crucial in healthcare, where the demand for services often fluctuates significantly. Big data facilitates the analysis of patient admissions, treatment patterns, and resource utilization, allowing healthcare administrators to make informed decisions on equipment and staff allocation. By analyzing historical data trends, hospitals can identify peak periods and allocate resources accordingly.For instance, a hospital equipped with big data analytics can:
- Track inventory levels of medical supplies and equipment in real-time, minimizing waste and ensuring availability.
- Utilize data to predict when specific resources will be needed, allowing for proactive ordering and staffing adjustments.
- Analyze patient flow and treatment times, optimizing bed occupancy rates and reducing patient wait times.
Implementing data-driven approaches in resource management not only enhances efficiency but also significantly impacts the overall patient experience.
Improvements in Scheduling and Staff Allocation
Big data provides healthcare facilities with the capability to optimize scheduling and staff allocation by analyzing patient patterns and staff performance metrics. This optimization leads to a more balanced workload, reducing burnout among healthcare professionals while improving care delivery.The importance of targeted staff allocation can be illustrated through the following:
- Predictive modeling helps forecast patient volumes, enabling hospitals to schedule the right number of staff during peak hours.
- Analyzing staff productivity data allows for the identification of high-performing employees and the tailoring of schedules to optimize their contributions.
- Advanced scheduling systems powered by data can adjust in real time based on unexpected patient surges or staff absences, ensuring continuous care.
Such improvements lead to increased staff satisfaction and decreased turnover rates, further enhancing operational efficiency.
Impact of Predictive Analytics on Emergency Room Operations
In emergency departments, where timely decision-making is critical, predictive analytics plays a vital role in managing patient inflow and resource allocation. By analyzing historical data, hospitals can predict peak times and types of emergencies, allowing for better preparation and response plans.The implementation of predictive analytics in emergency rooms can result in:
- Improved triage processes by identifying high-risk patients who require immediate attention, thus reducing the likelihood of adverse outcomes.
- Enhanced patient throughput by anticipating demand and optimizing staff and bed availability accordingly.
- Data-driven insights into the effectiveness of treatment protocols and patient outcomes, leading to continuous improvement in care delivery.
By leveraging predictive analytics, emergency departments can operate more efficiently, ultimately saving lives and reducing operational costs.
Research and Development in Healthcare
Big data is redefining the landscape of research and development in the healthcare sector. By harnessing vast amounts of health-related data, researchers can uncover insights that significantly enhance the drug discovery process, optimize clinical trials, and ultimately lead to more effective health interventions. This transformative capability not only accelerates innovation but also improves patient outcomes by ensuring that treatments are based on evidence gathered from comprehensive data analysis.
Acceleration of Drug Discovery Processes
The integration of big data analytics in drug discovery processes has revolutionized how pharmaceutical companies identify and develop new medications. This approach enables the analysis of diverse datasets, including genetic information, clinical trial data, and even patient demographics, to identify potential drug candidates more efficiently.
Genomic Data Analysis
By utilizing genomic data, researchers can identify genetic markers associated with specific diseases, allowing for the target-based drug discovery approach. This has led to the development of personalized medicine, where treatments are tailored to individual genetic profiles.
Predictive Analytics
Big data tools enable predictive modeling to anticipate how compounds will react in the human body, significantly reducing the time and cost associated with laboratory experiments.
Real-World Evidence
The use of electronic health records and patient registries provides invaluable real-world evidence that can inform drug efficacy and safety, leading to more informed decision-making in the drug development pipeline.
Clinical Trial Management and Participant Selection
Big data plays a pivotal role in enhancing the management of clinical trials, particularly in optimizing participant selection and improving overall trial efficiency.
Performance issues can arise in databases due to various factors, leading to frustrating bottlenecks. Knowing what are the most common database performance bottlenecks allows database administrators to proactively address these challenges, ensuring smoother operations and better user experience across applications.
Patient Recruitment
By analyzing electronic health records, researchers can efficiently identify and recruit eligible patients who meet specific criteria, significantly reducing the time needed for participant enrollment.
Site Selection
Advanced analytics can pinpoint clinical trial sites with the highest potential for recruitment based on historical data and patient demographics, thus maximizing resource allocation.
Trial Monitoring
Continuous monitoring of patient data during trials facilitates real-time adjustments to protocols and enhances patient safety, leading to more robust trial outcomes.
Case Studies of Successful Health Interventions Driven by Big Data Insights
Numerous successful health interventions have been enabled by the insights gained through big data analytics, showcasing its potential to transform healthcare delivery.
IBM Watson and Oncology
IBM Watson has implemented big data analytics to assist oncologists in diagnosing and recommending treatment plans based on a comprehensive review of patient data and research literature. This approach has led to more personalized treatment options for cancer patients, improving outcomes and survival rates.
Cleveland Clinic’s Genomic Medicine Institute
The institute utilizes big data to analyze genetic data in conjunction with clinical data to provide personalized treatment plans for patients with genetic disorders. This initiative has significantly advanced the understanding of complex diseases and has led to targeted therapies.
Google DeepMind and NHS
In partnership with the NHS, Google DeepMind has developed algorithms that analyze medical images to assist in the early detection of conditions such as diabetic retinopathy. This intervention has the potential to prevent blindness in high-risk patients and illustrates the power of machine learning in healthcare.These advancements underscore the critical role that big data continues to play in advancing research and development in healthcare, ultimately leading to innovative solutions that improve patient care and enhance the efficiency of healthcare systems.
Public Health and Epidemiology: Big Data Applications In Healthcare Industry
Big data is transforming the landscape of public health and epidemiology, providing invaluable insights that enhance our ability to manage and respond to health crises. By leveraging vast datasets from various sources, health organizations can track disease outbreaks, identify trends, and formulate effective health policies. This data-driven approach not only aids in immediate response efforts but also lays the groundwork for long-term public health strategies.Big data plays a pivotal role in monitoring and analyzing health trends and disease outbreaks.
The integration of data from health records, social media, environmental sensors, and genomic sequences allows health officials to detect patterns and predict the spread of diseases. For instance, during the Ebola outbreak in West Africa, big data analytics helped track the movement of the virus and the effectiveness of containment measures. By analyzing real-time data, authorities were able to implement targeted interventions, ultimately saving lives and resources.
Tracking Disease Outbreaks and Public Health Trends, Big data applications in healthcare industry
The application of big data in tracking disease outbreaks extends beyond mere surveillance; it is crucial for timely intervention and resource allocation. Various methodologies are employed to analyze data effectively:
- Real-time Surveillance: Continuous monitoring of health data, including hospital records and emergency room visits, allows for the rapid identification of unusual health trends, such as spikes in influenza cases preceding the flu season.
- Predictive Modeling: Utilizing machine learning algorithms, health agencies can forecast potential outbreaks by analyzing factors like weather patterns, population movement, and historical data.
- Geospatial Analysis: Mapping disease prevalence geographically helps identify hotspots and demographics at risk, thereby guiding targeted public health responses.
Implementing these strategies not only enhances preparedness but also fosters a proactive approach in managing public health.
Identifying At-risk Populations and Preventive Measures
Big data analytics is instrumental in identifying populations at risk for various health conditions, enabling the formulation of tailored preventive measures. By analyzing demographics, socioeconomic factors, and historical health data, public health officials can prioritize resources and interventions. Key applications include:
- Health Risk Assessment: Data from electronic health records (EHRs) allows for stratifying populations based on risk factors such as age, pre-existing conditions, and lifestyle choices.
- Targeted Interventions: Programs designed to address specific health issues, such as smoking cessation for high-risk communities, can be developed based on data insights.
- Community Health Initiatives: Data-driven initiatives, such as vaccination drives in underserved areas, can effectively reduce health disparities.
Through these methods, public health agencies can enhance health outcomes and mitigate risks before they escalate into more serious public health concerns.
Correlation Between Big Data Analytics and Health Policy Formulation
The intersection of big data and health policy is increasingly significant as it empowers policymakers with empirical evidence to make informed decisions. By analyzing vast amounts of health information, decision-makers can better understand the effectiveness of existing policies and identify areas for improvement. Considerable aspects include:
- Evidence-based Policy Making: Policymakers utilize analytics to evaluate the impact of health initiatives, ensuring funding and resources are allocated effectively.
- Resource Allocation: Data insights guide where to deploy health resources, such as clinics or outreach programs, based on the needs of specific populations.
- Cross-sector Collaboration: Insights from big data facilitate cooperation among health sectors, government agencies, and community organizations, encouraging comprehensive public health strategies.
The evidence garnered from big data analytics not only enhances the effectiveness of health policies but also promotes accountability and transparency in public health initiatives.
“The integration of big data in public health enhances our ability to respond to and prevent health crises through informed decision-making.”
Patient Engagement and Satisfaction
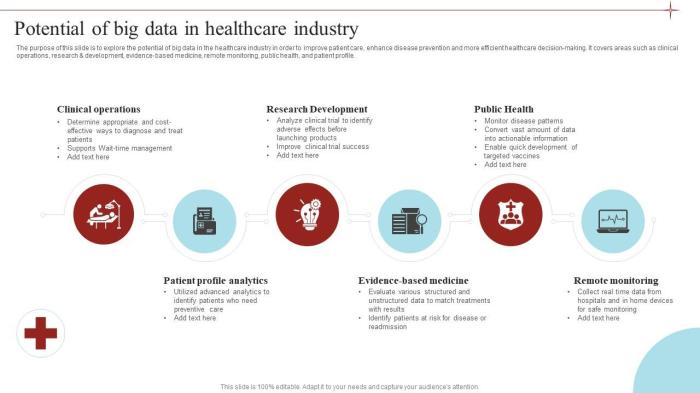
In today’s healthcare landscape, enhancing patient engagement and satisfaction is pivotal for not only improving clinical outcomes but also for fostering a positive patient experience. Big data plays a crucial role in this transformation by enabling healthcare providers to understand patient needs, preferences, and behaviors at a granular level. By leveraging the insights derived from big data, organizations can create personalized experiences that significantly enhance patient interactions with the healthcare system.One of the most effective strategies for leveraging big data to enhance patient experience is the implementation of personalized communication channels.
With the vast amount of data collected through electronic health records (EHRs), wearables, and patient surveys, healthcare providers can segment their patient populations to deliver tailored messages. This can include appointment reminders, medication adherence tips, and health management advice that resonates with individual patient circumstances.
Patient Portals Utilizing Big Data for Better Communication
Patient portals have emerged as a vital tool for enhancing communication between healthcare providers and patients. These platforms utilize big data analytics to streamline interactions and improve patient engagement. Key features of patient portals include:
- Access to Health Records: Patients can view their medical histories, lab results, and treatment plans, empowering them to take charge of their health.
- Secure Messaging: Secure channels allow patients to communicate directly with their healthcare providers, enabling quick responses to queries and concerns.
- Appointment Scheduling: Patients can conveniently book, reschedule, or cancel appointments online, reducing administrative burdens on healthcare staff.
- Personalized Health Insights: Utilizing data analytics, portals can provide tailored health recommendations based on individual patient data, leading to better adherence to treatment plans.
The integration of big data in these portals not only enhances communication but also fosters a sense of partnership between patients and healthcare providers.
Analyzing Patient Feedback and Improving Healthcare Services
Analyzing patient feedback is essential for continuously improving healthcare services. Big data analytics enables healthcare providers to gather and evaluate patient experiences through various channels such as surveys, online reviews, and social media. This information can be organized and assessed to identify common themes and areas for improvement.To effectively analyze patient feedback, healthcare organizations can employ the following methods:
- Sentiment Analysis: Advanced analytics techniques can gauge patient sentiment from feedback sources, highlighting areas of satisfaction and concern.
- Trend Identification: By examining feedback over time, healthcare providers can identify trends in patient experiences that may require immediate attention or long-term strategy adjustments.
- Benchmarking: Comparing patient feedback metrics against industry standards helps organizations understand their performance relative to competitors.
- Actionable Insights: Data visualization tools can convert complex feedback data into easily interpretable formats, allowing staff to make informed decisions swiftly.
By systematically analyzing patient feedback, healthcare organizations can implement targeted improvements that enhance service delivery and elevate overall patient satisfaction, ultimately leading to better health outcomes and loyalty.