Big data trends to watch in upcoming years are shaping the future of technology and business. The rapid evolution of fields like artificial intelligence, blockchain, and the Internet of Things (IoT) is transforming how organizations leverage vast amounts of data. As these innovations gain traction, they bring both exciting opportunities and complex challenges that require a deeper understanding of data privacy, governance, and industry-specific applications.
Emerging technologies are not only enhancing data analytics but also revolutionizing data security and integrity. As we explore the implications of these trends, it becomes evident that organizations must adapt to maintain compliance with evolving regulations and ensure ethical data usage. Understanding the diverse ways industries, from healthcare to retail, harness big data will be crucial in navigating this dynamic landscape.
Emerging Technologies Influencing Big Data
The landscape of big data analytics is continuously evolving, driven by groundbreaking technologies that enhance data processing, security, and generation capabilities. Understanding the intersection of these emerging technologies with big data is essential for grasping how businesses can leverage data for strategic advantages in the coming years.
Impact of Artificial Intelligence on Big Data Analytics
Artificial intelligence (AI) has transformed big data analytics by enabling more sophisticated data processing and analysis techniques. AI algorithms, particularly machine learning, can analyze vast datasets quickly and accurately, identifying patterns and trends that would take humans significantly longer to discern. This not only accelerates decision-making but also enhances predictive analytics, allowing organizations to forecast future trends with greater accuracy.
For instance, companies like Netflix utilize AI to analyze user data and viewing habits, subsequently providing personalized content recommendations. This not only improves user engagement but also drives revenue growth through targeted marketing strategies.
Influence of Blockchain Technology on Data Security and Integrity
Blockchain technology is revolutionizing data security and integrity in big data analytics by providing a decentralized method of storing and sharing information. By utilizing cryptographic techniques, blockchain ensures that data remains immutable and readily verifiable, effectively reducing the risk of data breaches and unauthorized access. The transparency offered by blockchain technology is particularly beneficial in industries such as finance and supply chain management.
For example, IBM’s Food Trust blockchain allows companies to track food products through the supply chain, ensuring transparency and safety, and enabling quick responses to potential contamination issues.
As technology evolves, the future of big data analytics promises to be transformative. With advancements in artificial intelligence and machine learning, organizations can harness vast data sets more effectively. This shift not only enhances decision-making but also fosters innovation, allowing businesses to stay competitive in an increasingly data-driven landscape.
Role of Internet of Things (IoT) Devices in Generating Big Data
The proliferation of Internet of Things (IoT) devices is a significant driver of big data generation. IoT devices, from smart home appliances to industrial sensors, constantly collect and transmit data, contributing to the enormous volumes of data that organizations must manage and analyze. This influx of data allows businesses to gain real-time insights into operations, customer behavior, and market trends.
For example, smart meters in energy consumption analytics provide utilities with data on electricity usage patterns, enabling better demand forecasting and resource allocation. The combination of IoT data with advanced analytics leads to improved operational efficiency and innovative business models that capitalize on real-time insights.
Data Privacy and Governance Trends
The landscape of big data is evolving rapidly, making data privacy and governance more critical than ever. Organizations are increasingly recognizing that effective data governance not only protects sensitive information but also enhances operational efficiency and builds consumer trust. In the coming years, navigating the complexities of data privacy will require businesses to implement robust governance frameworks that align with global regulations.Data governance plays a pivotal role in big data initiatives by establishing clear policies and processes for data management.
This includes data quality, accessibility, and security, all of which are vital for maintaining compliance with evolving regulations. A well-structured data governance program helps organizations mitigate risk, ensures accountability, and promotes ethical data handling practices.
Compliance with Global Data Protection Regulations
Adhering to global data protection regulations is essential for organizations that handle large volumes of data. Key regulations include the General Data Protection Regulation (GDPR) in Europe, the California Consumer Privacy Act (CCPA), and various others worldwide. Compliance can be achieved through a combination of strategies:
Data Mapping and Inventory
Organizations should create a comprehensive inventory of the data they collect, process, and store. This includes understanding data sources, types, locations, and any third-party involvement.
Risk Assessment
Conducting regular risk assessments helps identify vulnerabilities and areas of non-compliance. This proactive approach allows organizations to address issues before they become significant problems.
Identifying the most common database performance bottlenecks is crucial for optimizing your systems. Issues like slow queries, inefficient indexing, and hardware limitations can severely impact performance. Understanding these factors enables businesses to implement effective strategies to enhance efficiency and user experience, ensuring that data management processes run smoothly and responsively.
Regular Audits
Implementing periodic audits ensures that data governance policies are followed and that the organization remains compliant with the latest regulations.
Training and Awareness
Educating employees on data privacy principles and the importance of compliance fosters a culture of accountability and vigilance regarding data protection.
User Consent Management and Data Ownership Trends
Trends in user consent management are evolving as consumers demand greater control over their personal data. Organizations are shifting towards transparent data practices that empower users to make informed decisions. Key trends include:
Dynamic Consent Management
Businesses are adopting dynamic consent management systems that allow users to modify their consent preferences easily. This flexibility ensures that organizations remain compliant with laws requiring explicit consent for data processing.
Decentralized Identity Solutions
The rise of decentralized identity frameworks provides users with more control over their data. These solutions enable individuals to manage their identities without relying on a central authority, promoting data ownership and privacy.
Enhanced Transparency
Companies are increasingly focusing on transparency by clearly communicating how data is collected, used, and shared. This approach helps build trust and fosters a better relationship with customers.
User-Centric Regulations
Regulatory bodies are shifting towards user-centric laws, emphasizing the need for organizations to prioritize user rights and data protection.As businesses navigate the complexities of data governance and privacy, staying informed about these trends will be crucial for maintaining compliance and fostering trust with consumers.
Big Data in Various Industries: Big Data Trends To Watch In Upcoming Years
Big data is revolutionizing a multitude of industries, enhancing decision-making processes, operational efficiencies, and customer experiences. As organizations harness vast amounts of data, the implications for sectors like healthcare, retail, finance, and manufacturing are profound. Each industry is finding innovative applications for big data, showcasing its versatility and importance in today’s data-driven landscape.
Big Data in Healthcare
The healthcare sector is increasingly leveraging big data to improve patient outcomes and enhance operational efficiencies. By analyzing vast datasets from electronic health records, wearable devices, and clinical trials, healthcare providers can identify trends, predict patient needs, and personalize treatment plans.
- Predictive Analytics: Hospitals are employing predictive analytics to foresee patient admissions, enabling better resource allocation and reducing wait times.
- Population Health Management: Big data allows for the tracking of health trends across populations, aiding in the identification of at-risk groups and tailoring interventions accordingly.
- Drug Development: Pharmaceutical companies utilize big data to accelerate drug discovery by analyzing chemical compounds, genomic data, and clinical trial results.
Big Data in Retail
Retailers are harnessing big data to analyze consumer behavior, optimize inventory, and enhance customer engagement. By examining purchase histories, customer preferences, and market trends, businesses can make informed decisions that drive sales.
- Personalization: Retailers use big data to deliver targeted marketing campaigns tailored to individual customer preferences, enhancing the shopping experience.
- Inventory Management: Analyzing purchasing patterns helps retailers maintain optimal stock levels, reducing both overstock and stockouts.
- Customer Insights: Big data enables retailers to segment customers based on behavior, allowing for more effective promotions and product recommendations.
Big Data in Finance vs. Manufacturing
The applications of big data in finance and manufacturing showcase distinct yet transformative impacts within these sectors.
- Finance: Financial institutions use big data for risk management, fraud detection, and personalized banking services. Analyzing transaction data in real-time helps detect anomalies, thus preventing fraudulent activities.
- Manufacturing: In manufacturing, big data drives efficiencies through predictive maintenance, supply chain optimization, and quality control. Sensors embedded in machinery collect data, enabling real-time analysis that predicts failures before they occur, minimizing downtime.
“Big data is the new oil; it drives innovation and efficiency across industries.” – Unknown
Future Predictions for Big Data Analytics
The landscape of big data analytics is poised for transformative advancements in the coming years. As organizations increasingly rely on data-driven decisions, the methodologies and technologies supporting predictive analytics will evolve significantly. This section explores anticipated advancements, expected challenges, and the future of real-time data processing, providing a comprehensive view of the trajectory of big data analytics.
Advancements in Predictive Analytics Techniques
The future of predictive analytics is likely to feature several key advancements that will enhance the accuracy and efficiency of data interpretation. These advancements are expected to leverage machine learning, artificial intelligence, and advanced statistical methods to derive actionable insights from vast data sets.
- Enhanced Machine Learning Algorithms: Future predictive analytics will incorporate more sophisticated machine learning algorithms, enabling models to learn from data at an accelerated pace. For instance, techniques such as deep learning are expected to significantly improve the accuracy of predictions across various sectors, including finance and healthcare.
- Automated Data Preparation: Automation in data cleansing and preparation will facilitate quicker analysis and reduce the time spent in preprocessing steps. Tools that automatically identify and rectify data anomalies will be crucial.
- Integration with Internet of Things (IoT): The fusion of IoT with predictive analytics will allow organizations to gather real-time data from connected devices, resulting in timely predictions that can inform decision-making processes.
- Natural Language Processing (NLP): The incorporation of NLP in predictive analytics will enable organizations to analyze unstructured data such as social media interactions, customer feedback, and reviews, thus enriching the contextual understanding of data.
Challenges Organizations Will Face
As big data technologies continue to evolve, organizations will encounter several challenges that could hinder their ability to fully leverage data analytics. These challenges stem from the complexity of managing large data sets, regulatory compliance, and the need for skilled personnel.
- Data Privacy Regulations: Compliance with evolving data privacy laws, such as GDPR and CCPA, will be a significant challenge. Organizations must ensure that their data collection and processing practices are transparent and secure.
- Data Quality and Integration: Maintaining the quality and integrity of data across multiple sources remains a persistent challenge. Integrating diverse data types while ensuring consistency will be crucial for predictive analytics success.
- Skill Shortages: The demand for data scientists and analysts is surpassing supply. Organizations will face difficulties in attracting and retaining talent necessary to leverage advanced analytics tools effectively.
- Security Threats: As reliance on data increases, so does the risk of data breaches and cyberattacks. Organizations must prioritize security measures to protect sensitive information.
Future of Real-Time Data Processing
The capability for real-time data processing is expected to reshape how organizations operate and interact with their stakeholders. With advancements in technology, such as edge computing and 5G connectivity, the implications for real-time analytics will be profound.Real-time data processing will enable organizations to make instantaneous decisions based on current conditions, enhancing operational efficiency and customer experience. For example, retailers can leverage real-time analytics to optimize inventory management and respond to consumer behavior swiftly.
“Real-time analytics will redefine competitive advantage, enabling organizations to respond to market changes and customer needs instantaneously.”
The integration of real-time analytics with artificial intelligence will allow for predictive capabilities that can forecast trends and behaviors in near real-time, providing organizations with a strategic edge. The evolution of big data technologies will thus create an environment where rapid responsiveness and proactive decision-making become the cornerstone of successful business strategies.
Skills and Workforce Development for Big Data
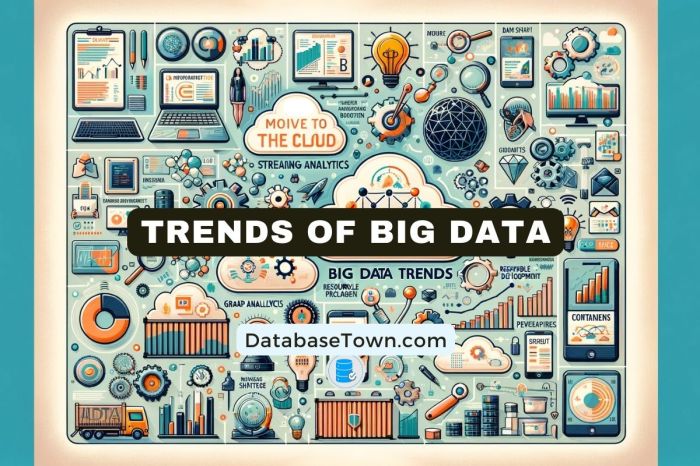
In today’s data-driven landscape, the demand for skilled professionals in big data analytics is at an all-time high. Organizations are recognizing that to harness the power of big data, they need a workforce equipped with the right skills. This segment explores the essential skills required for professionals in big data roles, strategies for upskilling the workforce, and the critical role of interdisciplinary teams in successfully executing big data projects.
Key Skills for Big Data Professionals
To thrive in a big data environment, professionals must possess a blend of technical and soft skills. While technical expertise forms the foundation of any data role, soft skills enhance collaboration and communication within teams. The following are key skills needed:
- Data Analysis and Interpretation: Ability to analyze complex data sets and derive actionable insights is fundamental.
- Statistical Knowledge: Understanding statistical methods and machine learning algorithms enables professionals to create predictive models.
- Programming Skills: Proficiency in languages such as Python, R, and SQL is crucial for data manipulation and analysis.
- Data Visualization: Skills in tools like Tableau or Power BI help in presenting data findings effectively to stakeholders.
- Cloud Computing: Familiarity with cloud platforms (e.g., AWS, Azure) is increasingly important as organizations shift data storage and processing to the cloud.
- Soft Skills: Critical thinking, communication, and teamwork are essential for collaborating within diverse teams and conveying data insights clearly.
Strategies for Upskilling the Workforce, Big data trends to watch in upcoming years
Organizations must proactively invest in upskilling their workforce to keep pace with evolving big data technologies and methodologies. Successful strategies include:
- Continuous Learning Programs: Implementing regular training sessions and workshops to keep employees updated on the latest big data tools and techniques.
- Mentorship and Coaching: Pairing experienced data professionals with newer employees fosters knowledge transfer and skill enhancement.
- Online Courses and Certifications: Encouraging participation in reputable online courses from platforms like Coursera or edX can help staff gain new competencies efficiently.
- Cross-Departmental Training: Facilitating training that allows employees from different departments to learn about big data applications relevant to their fields enhances overall data literacy.
Importance of Interdisciplinary Teams
The complexity of big data projects necessitates collaboration across various disciplines, making interdisciplinary teams vital for success. These teams typically consist of data scientists, business analysts, IT specialists, and domain experts. The benefits of such collaboration include:
- Diverse Perspectives: Different expertise leads to innovative solutions and a holistic understanding of data challenges.
- Improved Problem-Solving: Teams can address multifaceted issues more effectively through shared knowledge and skills.
- Enhanced Communication: Interdisciplinary collaboration promotes clearer communication of data insights and business implications.
- Faster Decision-Making: Diverse teams can quickly assess data-driven recommendations from various angles, leading to timely and informed decisions.