Future of business intelligence in the digital age marks a transformative period where data is an invaluable asset driving decisions across industries. As organizations increasingly rely on data analytics to gain insights and formulate strategies, the integration of emerging technologies is reshaping how business intelligence operates. From advanced data visualization tools to predictive analytics, businesses are better equipped to forecast trends and make informed choices.
The landscape of business intelligence is evolving, influenced by artificial intelligence and machine learning, which enhance data processing and insights. The necessity for real-time data further underscores the competitive advantage it provides, allowing businesses to act swiftly and effectively in dynamic markets. However, the journey isn’t without challenges, as organizations must navigate data governance and resistance to change while embracing the future trends that will define this field.
The Role of Data Analytics in Business Intelligence
Data analytics serves as the backbone of business intelligence, enabling organizations to transform raw data into meaningful insights that drive strategic decision-making. In today’s fast-paced digital age, the effective use of data analytics not only enhances operational efficiency but also fosters a culture of informed decision-making, ultimately leading to competitive advantages.Data analytics empowers organizations by allowing decision-makers to base their strategies on empirical data, leading to more accurate forecasting and improved business outcomes.
Integrating business intelligence with existing systems can significantly enhance operational efficiency and data accuracy. It’s essential to ensure compatibility and seamless data flow between platforms, which ultimately supports better decision-making. To learn more about the integration process, read up on the steps outlined in this resource about how to integrate business intelligence with existing systems.
By leveraging various analytical techniques, businesses can uncover patterns, trends, and anomalies that might otherwise go unnoticed. This analytical prowess drives better business strategies and enhances overall performance.
Tools for Data Visualization in Business Intelligence
Effective data visualization is crucial for interpreting complex datasets and communicating insights clearly. Numerous tools have emerged that enhance the visualization of data, making it easier for stakeholders to grasp critical information at a glance. Here are some notable tools widely adopted in the industry:
- Tableau: Renowned for its user-friendly interface, Tableau allows users to create interactive and shareable dashboards. It enables businesses to visualize data intuitively, facilitating quick insights and better decision-making.
- Power BI: Developed by Microsoft, Power BI integrates with various data sources and offers robust visualization capabilities. Its drag-and-drop functionality makes it accessible for users with varying levels of technical expertise.
- QlikView: QlikView excels in associative data modeling, enabling users to explore data relationships dynamically. Its powerful visualization features allow organizations to create detailed reports that reveal hidden insights.
The adoption of these visualization tools enhances an organization’s ability to present data-driven insights effectively, ensuring that all stakeholders can engage with and understand the data being analyzed.
To make informed business intelligence decisions, it’s crucial to know how to analyze data effectively. Understanding the key metrics and trends is essential, and you can explore detailed insights on how to analyze data for business intelligence decisions to enhance your analytical skills. This will ultimately lead to better decision-making processes within your organization.
Benefits of Predictive Analytics in Forecasting Business Trends
Predictive analytics plays a pivotal role in shaping future business strategies by enabling organizations to anticipate market trends and customer behavior. As businesses increasingly rely on data-driven insights, the incorporation of predictive analytics provides several key advantages:
- Enhanced Forecasting Accuracy: Predictive analytics utilizes historical data and statistical algorithms to forecast future outcomes with greater precision. This capability allows businesses to make informed decisions based on probable scenarios rather than mere speculation.
- Proactive Risk Management: By identifying potential risks and opportunities in advance, organizations can mitigate challenges before they escalate. For instance, retailers can adjust inventory levels based on predicted demand, minimizing stockouts or excess inventory.
- Personalized Customer Experiences: Understanding customer behavior patterns enables companies to tailor their marketing efforts, leading to improved customer satisfaction and retention rates. For example, online retailers can use predictive models to recommend products that align with individual customer preferences.
Incorporating predictive analytics into business intelligence not only enhances decision-making but also equips organizations with the foresight needed to navigate the complexities of today’s dynamic market landscape.
Emerging Technologies Shaping Business Intelligence
The landscape of business intelligence (BI) is rapidly evolving, driven by the advent of emerging technologies. As organizations strive to harness the power of data, innovations such as artificial intelligence (AI), machine learning (ML), and cloud computing are revolutionizing the way businesses process, analyze, and utilize information. These technologies not only enhance decision-making but also empower organizations to remain competitive in the digital age.
Impact of Artificial Intelligence on Data Processing in Business
Artificial intelligence is transforming data processing by automating complex tasks that traditionally required human intervention. With AI, businesses can analyze vast amounts of data at unprecedented speeds, facilitating real-time insights. This capability allows organizations to make informed decisions based on predictive analytics and historical data trends. AI algorithms can identify patterns and anomalies, streamlining data management processes and reducing the likelihood of errors.
A notable example of AI-driven data processing is IBM’s Watson, which utilizes natural language processing to analyze unstructured data, enabling businesses to extract actionable insights. The integration of AI in BI not only improves efficiency but also provides a competitive edge through enhanced data-driven strategies.
Machine Learning Algorithms Improving Insights Derived from Data
Machine learning algorithms play a crucial role in enhancing the quality of insights derived from data. These algorithms learn from past data patterns to make predictions about future outcomes, thus enabling businesses to develop more accurate forecasting models. By continuously improving through experience, machine learning systems adapt to new data, refining their predictions over time.The application of machine learning in BI can be observed in various sectors.
For example, retail companies leverage recommendation engines that analyze customer behavior and preferences, driving personalized marketing strategies and increasing sales conversions. Additionally, financial institutions use machine learning for credit risk assessment, allowing for more precise loan approvals based on historical data trends and individual customer profiles.
Understanding how to analyze data for business intelligence decisions is crucial for any organization aiming to leverage information for strategic growth. By focusing on key metrics and utilizing appropriate tools, businesses can extract actionable insights that drive informed choices. For a deeper dive into effective techniques, check out this guide on how to analyze data for business intelligence decisions.
Cloud Computing Solutions Supporting Business Intelligence Functions
Cloud computing has become an indispensable component of modern business intelligence, providing scalable and flexible solutions for data storage and analysis. By migrating BI functions to the cloud, organizations can leverage advanced analytics tools without the need for extensive on-premise infrastructure. This shift not only reduces costs but also enhances collaboration across teams and locations.Several cloud-based BI platforms exemplify this trend.
For instance, Microsoft Power BI offers users the ability to create interactive reports and dashboards that can be accessed from anywhere, fostering real-time collaboration among stakeholders. Similarly, Tableau Online empowers organizations to visualize data and share insights effortlessly, enhancing the overall decision-making process.Moreover, cloud computing facilitates the integration of multiple data sources, enabling unified analytics that promote deeper insights and strategic planning.
As businesses continue to embrace cloud solutions, the potential for innovation in business intelligence is boundless.
The Importance of Real-Time Data in Decision Making
In today’s fast-paced digital landscape, the ability to harness real-time data has become a cornerstone for businesses striving to maintain a competitive edge. As organizations face increasingly dynamic markets, the immediacy of data insights can dramatically influence decision-making processes, allowing companies to respond swiftly to emerging trends and changes in consumer behavior.Real-time data analytics enables organizations to monitor key performance indicators and metrics as they happen, providing a comprehensive view of operations and market conditions.
This immediacy is crucial for identifying opportunities and threats, which can lead to informed strategies that enhance overall efficiency and drive growth. Businesses that prioritize real-time analytics gain a significant advantage over competitors who rely on traditional data reporting methods, which often lag in delivering actionable insights.
Comparison of Tools for Real-Time Analytics versus Traditional Methods
The distinction between real-time analytics tools and traditional reporting methods is critical for understanding their respective strengths. Real-time analytics tools provide instantaneous access to data, while traditional methods often involve periodic data updates. Here is a comparative overview of the two approaches:
Feature | Real-Time Analytics Tools | Traditional Reporting Methods |
---|---|---|
Data Update Frequency | Continuous | Scheduled (e.g., daily, weekly) |
Data Processing | Instantaneous processing of data streams | Batch processing with delayed insights |
Decision-Making Speed | Immediate adjustments and responses | Delayed responses due to outdated data |
Use Cases | Operational monitoring, fraud detection, real-time marketing | Monthly reports, historical analysis |
Real-time data analytics fosters an environment where businesses can pivot quickly in response to market fluctuations, providing a vital advantage in today’s competitive landscape.
Industries Benefiting from Real-Time Business Intelligence
Numerous industries have recognized the transformative power of real-time business intelligence, leveraging it to enhance performance and optimize operations. The following sectors are particularly well-suited to benefit from real-time analytics:
- Retail: Real-time inventory tracking and customer behavior analysis enable retailers to optimize stock levels and improve customer engagement.
- Healthcare: Real-time patient monitoring systems contribute to better patient outcomes and operational efficiency in medical facilities.
- Finance: Financial institutions utilize real-time data for fraud detection, risk management, and market analysis, allowing for timely investment decisions.
- Manufacturing: Real-time production data helps manufacturers identify bottlenecks and maintain optimal workflow, improving overall efficiency.
- Transportation and Logistics: Real-time tracking of shipments and fleet management enhances delivery efficiency and customer satisfaction.
- Telecommunications: Service providers use real-time analytics to monitor network performance and customer usage patterns, ensuring quality service delivery.
As industries increasingly adopt real-time data strategies, they position themselves for greater agility and responsiveness, driving better outcomes in an ever-evolving business landscape.
Challenges and Limitations in Business Intelligence Adoption
Organizations are increasingly recognizing the importance of business intelligence (BI) as a strategic asset in the digital age. Despite this growing recognition, many face significant challenges when adopting new BI systems. These challenges can impede the effective use of data, leading to suboptimal decision-making and reduced competitive advantage. Addressing these obstacles is crucial for fully leveraging the potential of BI.One of the primary challenges organizations encounter is resistance to change.
Employees may be accustomed to existing processes and can be hesitant to adopt new technologies that alter their workflows. Additionally, technical challenges, such as integrating BI tools with legacy systems or managing vast amounts of data, often arise. Furthermore, data quality issues can undermine the effectiveness of BI initiatives, making it imperative for organizations to establish robust data governance frameworks.
Obstacles in Adopting Business Intelligence Systems
Organizations face several common obstacles that can hinder the successful adoption of BI systems. Understanding these challenges allows businesses to develop targeted strategies for effective implementation. Key obstacles include:
- Resistance to Change: Employees may resist new systems due to fear of the unknown or perceived threats to job security.
- Integration Issues: Difficulty in integrating BI tools with existing systems can lead to data silos and hinder analysis.
- Data Quality Concerns: Inaccurate or incomplete data can compromise the reliability of BI insights, making it crucial to address data quality upfront.
- Cost Constraints: The financial investment required for BI systems can be a barrier, especially for smaller organizations.
- Lack of Expertise: Insufficient training and knowledge among staff can limit the effective use of BI tools, necessitating ongoing education initiatives.
Importance of Data Governance in Maintaining Data Quality
Data governance is a critical component of successful BI adoption, ensuring that data remains accurate, consistent, and secure. An effective governance framework helps organizations to establish policies and standards for data management, fostering trust in the integrity of the data used for decision-making. Implementing a robust data governance strategy involves:
- Establishing Clear Policies: Defining data ownership, access controls, and usage guidelines to promote accountability.
- Regular Data Audits: Conducting audits to identify and rectify data quality issues, ensuring the accuracy and reliability of information.
- Training and Awareness: Educating employees on the importance of data governance and best practices for data management.
- Implementing Data Stewardship: Appointing data stewards who oversee data quality and governance initiatives across the organization.
Strategies for Overcoming Resistance to Change, Future of business intelligence in the digital age
Overcoming resistance to change is essential for the successful adoption of BI systems. Organizations can employ several strategies to facilitate a smoother transition:
- Involvement in Decision-Making: Engaging employees in the decision-making process increases buy-in and reduces resistance.
- Effective Communication: Clearly communicating the benefits of BI systems can help alleviate fears and encourage acceptance.
- Providing Training: Offering comprehensive training programs empowers employees to use new tools confidently and competently.
- Showcasing Quick Wins: Demonstrating early successes from BI initiatives can foster enthusiasm and support among staff.
Future Trends in Business Intelligence: Future Of Business Intelligence In The Digital Age
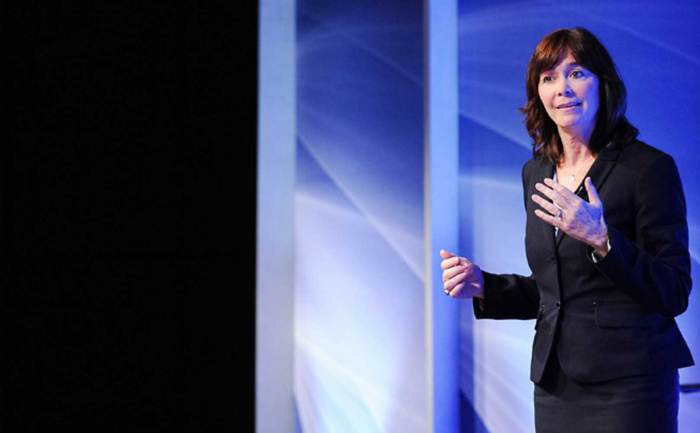
As we venture into the next decade, the landscape of business intelligence (BI) is poised for significant transformation. The role of data continues to expand, influenced by evolving technologies, regulatory frameworks, and changing consumer behaviors. This segment explores key predictions surrounding the future of BI, the critical importance of data privacy and ethics, and the profound impact of the Internet of Things (IoT) on data practices.
Predictions for the Evolution of Business Intelligence
The future of business intelligence will be characterized by greater integration of advanced technologies and enhanced user experiences. Organizations are expected to leverage AI-driven analytics, which will allow for more sophisticated data interpretation and forecasting. Key predictions include:
- Increased Automation: Automation will streamline data collection and analysis processes, reducing the need for manual intervention. Businesses will utilize advanced algorithms to enhance decision-making speed and accuracy.
- Enhanced Predictive Analytics: The use of predictive analytics will rise, enabling organizations to anticipate market trends, customer preferences, and operational challenges effectively.
- Self-Service BI Tools: A shift towards self-service business intelligence tools will empower non-technical users to generate insights independently, democratizing data access across organizations.
- Cloud-Based Solutions: The transition to cloud-based BI solutions will continue, offering scalability, flexibility, and cost-effectiveness. This will facilitate real-time data access and collaboration among distributed teams.
Significance of Data Privacy and Ethics in Business Intelligence
As data collection and analysis become ubiquitous, the importance of data privacy and ethical practices in business intelligence will intensify. Organizations must navigate a complex landscape of regulations, such as GDPR and CCPA, while fostering trust with their customers. Key aspects include:
- Regulatory Compliance: Adherence to data protection regulations will be crucial for businesses, necessitating robust data governance frameworks to manage and safeguard customer information.
- Transparent Data Usage: Companies will be expected to be transparent about how they collect, store, and utilize data, promoting ethical data practices that align with consumer expectations.
- Data Security Measures: Investments in advanced cybersecurity protocols will be essential to protect sensitive information from breaches, ensuring that personal data remains safe and secure.
- Ethical AI Use: The ethical implications of AI in BI will come under greater scrutiny, prompting organizations to establish guidelines that prevent bias and ensure fairness in analytics.
Impact of the Internet of Things on Data Collection and Analysis
The rise of the Internet of Things (IoT) will profoundly influence data collection and analysis for businesses. As IoT devices proliferate, they will generate vast amounts of data, enhancing the capabilities of business intelligence systems. Notable impacts include:
- Real-Time Data Gathering: IoT devices will enable real-time data collection across various sectors, providing businesses with immediate insights into operational performance and customer behavior.
- Enhanced Customer Insights: Businesses will gain deeper insights into customer preferences and usage patterns through data collected from IoT devices, informing product development and marketing strategies.
- Operational Efficiency: The integration of IoT in operations will lead to improved efficiency, as businesses can monitor processes and equipment in real time, reducing downtime and optimizing resource allocation.
- New Business Models: The data generated by IoT will pave the way for innovative business models, including subscription-based services and predictive maintenance offers, fundamentally changing how businesses interact with consumers.