How big data impacts decision making is a crucial topic in today’s data-driven landscape, where organizations leverage vast amounts of information to refine their strategies and improve outcomes. The sheer volume and variety of data available today enable businesses to make informed decisions that were previously based on intuition alone. By understanding the characteristics and sources of big data, along with the technologies that gather and analyze it, organizations can unlock significant value and enhance their decision-making processes.
This exploration delves into how big data reshapes strategic choices, the types of decisions that benefit from data-driven insights, and the tools that facilitate this analysis. With real-world examples and case studies, we will highlight the transformative power of big data in various industries and Artikel the future trends that may further influence decision-making paradigms.
Understanding Big Data
Big data refers to the vast volumes of structured and unstructured data generated by various sources and processed for actionable insights. As organizations increasingly rely on data to drive decisions, understanding the nature and characteristics of big data becomes crucial. It encompasses not only the size of the data but also its variety, velocity, and veracity, which together form the foundation for effective data analysis and utilization.Big data can be characterized by the “Four V’s”: volume, variety, velocity, and veracity.
Volume pertains to the sheer amount of data generated, which can be in petabytes or even exabytes. Variety addresses the different types of data—structured, semi-structured, and unstructured—arising from various sources. Velocity refers to the rapid speed at which data is generated and must be analyzed for optimal decision-making, while veracity denotes the quality and reliability of the data.
When it comes to maintaining optimal database performance, utilizing the right tools is crucial. The Best tools for database monitoring and performance analysis can help you identify bottlenecks and ensure your databases run smoothly. These tools offer insights that allow for proactive management, ultimately leading to a more efficient data environment.
Sources of Big Data in Modern Organizations
In today’s digital landscape, big data is sourced from a multitude of channels, providing organizations with a comprehensive view of their operations and customer interactions. Understanding these sources is vital for organizations aiming to leverage big data effectively.Key sources of big data include:
- Social Media: Platforms like Facebook, Twitter, and Instagram generate massive amounts of data through user interactions, posts, and shared content, serving as a rich source of consumer sentiment and behavior.
- Internet of Things (IoT): Devices connected to the internet, such as smart appliances and wearables, continuously collect and transmit data, contributing to the growing landscape of big data.
- Transactional Data: Businesses generate vast amounts of data from sales transactions, customer interactions, and operational processes, which can be analyzed to identify trends and improve efficiency.
- Web and Mobile Analytics: Data collected from website traffic and mobile app usage provides insights into user behavior and preferences, aiding in targeted marketing efforts.
- Public Data: Government databases and open data initiatives contribute valuable information that organizations can utilize for research and analysis.
Role of Data Collection Technologies in Gathering Big Data
Data collection technologies play a pivotal role in harnessing big data from its various sources. These technologies not only facilitate the gathering of data but also ensure its integrity and usability for analysis.The importance of these technologies is underscored by the following tools and methods:
- Data Warehousing: Centralized repositories that store large volumes of structured data from different sources, allowing for efficient retrieval and analysis.
- Cloud Computing: Provides scalable storage solutions and processing power necessary for handling big data, enabling organizations to access resources on-demand.
- Data Lakes: These are storage repositories that hold vast amounts of raw data in its native format until it is needed for analysis, allowing for flexibility in processing.
- Data Streaming Technologies: Tools like Apache Kafka and AWS Kinesis enable real-time data processing and analysis, essential for industries that rely on instant insights.
- Machine Learning Algorithms: These are utilized to analyze large datasets, uncover patterns, and generate predictive insights, optimizing decision-making processes.
“The true potential of big data lies in the ability to derive meaningful insights that drive strategic decisions.”
The Role of Big Data in Decision-Making
Big data has emerged as a transformative force in the realm of decision-making, significantly influencing how organizations strategize and operate across various sectors. In an era dominated by information, the ability to glean actionable insights from vast datasets allows businesses to navigate complexities and seize opportunities with unprecedented precision. By leveraging analytics, companies can enhance their strategic planning and execution, ensuring decisions are not solely based on intuition but are driven by evidence and analytics.The influence of big data on strategic decision-making processes is profound.
Database query optimization is essential for improving performance and efficiency. Common techniques include indexing, which speeds up data retrieval, and query rewriting to minimize complexity. For a deeper understanding of these methods, check out the article on What are the common database query optimization techniques. Implementing these strategies can significantly enhance your database operations.
Organizations utilize data to identify trends, forecast outcomes, and refine their strategies, making the decision-making process more robust and data-centric. By analyzing historical data alongside current metrics, businesses can develop a clearer understanding of market dynamics, consumer behavior, and operational efficiency. This empirical approach minimizes the risks associated with decision-making and ensures that strategies align closely with real-world conditions.
Types of Decisions Enhanced by Big Data Insights
Incorporating big data into decision-making enhances various types of decisions within an organization. The context of these decisions often dictates the specific data utilized, but common categories include:
- Operational Decisions: Data analytics streamline processes, optimize supply chains, and improve resource allocation, leading to increased efficiency and reduced costs.
- Marketing Decisions: By analyzing customer data, organizations can personalize marketing campaigns, target specific demographics, and predict customer preferences to improve engagement and conversion rates.
- Financial Decisions: Big data informs investment strategies, risk management, and financial forecasting, enabling companies to make informed decisions about capital allocation.
- Product Development Decisions: Insights from consumer feedback and market trends guide organizations in designing and launching products that meet customer needs, thereby reducing time-to-market and enhancing competitiveness.
Data-driven decisions starkly contrast with intuition-based decisions. While the latter relies heavily on gut feelings and personal experience, data-driven decision-making is grounded in quantitative analysis and empirical evidence. For instance, a retailer may historically have adjusted inventory levels based on anecdotal evidence from sales staff. In contrast, leveraging big data analytics allows the retailer to analyze purchasing patterns, seasonality, and external factors, providing a comprehensive view that informs inventory management.
“The transition from intuition to data-driven decisions not only enhances accuracy but also empowers organizations to adapt swiftly to changing market conditions.”
In practical terms, a company faced with declining sales may have traditionally relied on intuition to redirect its marketing strategy. However, a big data approach would involve analyzing customer purchasing trends, social media sentiment, and competitive benchmarks, leading to a more informed pivot in strategy. This shift reduces the likelihood of costly missteps and enhances overall organizational agility, ultimately contributing to sustained growth and competitiveness in the marketplace.
Tools and Technologies for Big Data Analysis: How Big Data Impacts Decision Making
The landscape of big data analysis is underpinned by a variety of sophisticated tools and technologies designed to handle vast datasets. Organizations increasingly rely on these resources to derive insights that influence strategic decisions. Understanding the capabilities and limitations of these tools is essential for maximizing their potential in decision-making processes.
Popular Big Data Tools and Platforms, How big data impacts decision making
A multitude of tools and platforms is available in the big data ecosystem, each offering unique features tailored to different analytical needs. Some of the most prominent tools include:
- Apache Hadoop: An open-source framework that enables distributed storage and processing of large datasets across clusters of computers using simple programming models. It is highly scalable and fault-tolerant.
- Apache Spark: Known for its speed and ease of use, Spark allows for in-memory data processing, significantly improving the performance of big data applications. It supports various programming languages, including Java, Python, and Scala.
- NoSQL Databases (e.g., MongoDB, Cassandra): These databases are designed to handle unstructured data and provide flexible data models, making them ideal for big data applications that require horizontal scaling.
- Tableau: A powerful data visualization tool that enables users to create interactive and shareable dashboards with real-time data insights, making complex data more accessible and understandable.
- Apache Kafka: A distributed streaming platform capable of handling real-time data feeds, making it a crucial tool for building real-time analytical systems and integrating big data solutions.
Comparison of Big Data Technologies
When evaluating different big data technologies, factors such as usability and efficiency play a critical role in determining the best fit for specific business needs. The following table provides a comparison of select big data tools based on key criteria:
Tool | Usability | Efficiency | Scalability |
---|---|---|---|
Apache Hadoop | Moderate learning curve | High for batch processing | Excellent |
Apache Spark | Low learning curve | Very high for both batch and stream processing | Good |
NoSQL Databases | Varies by implementation | High for unstructured data | Excellent |
Tableau | Very user-friendly | High for visualization | Limited for data processing |
Apache Kafka | Moderate learning curve | High for real-time data processing | Excellent |
Best Practices for Implementing Big Data Solutions
Implementing big data solutions effectively requires adherence to certain best practices that enhance the likelihood of achieving desired outcomes. These practices include:
- Define clear objectives: Establish what specific questions you aim to answer with your data analysis.
- Invest in training: Provide training for staff to ensure they understand how to use data tools effectively.
- Focus on data quality: Ensure data is accurate, complete, and consistent to derive reliable insights.
- Establish a strong data governance framework: Implement policies and procedures to manage data access and usability securely.
- Iterate and optimize: Regularly review and refine data processes and tools based on performance feedback and evolving business needs.
Effective implementation of big data solutions not only enhances decision-making capabilities but also drives innovation and competitive advantage.
Case Studies of Big Data in Action
The implementation of big data analytics has revolutionized the way organizations approach decision-making. By leveraging vast amounts of data, companies across various industries are not only improving their operational efficiency but also enhancing customer experience and driving innovation. This section explores notable examples of businesses that have successfully integrated big data into their strategies, illustrating the diverse applications and benefits it offers.
Successful Examples of Big Data Utilization
Numerous companies have harnessed big data to gain a competitive edge. These examples demonstrate the transformative power of data in decision-making processes across different sectors.
- Netflix: The streaming giant utilizes big data analytics to tailor content recommendations to individual users. By analyzing viewing patterns, preferences, and demographic data, Netflix ensures user engagement, significantly boosting retention rates and subscription growth.
- Amazon: Amazon’s recommendation engine is another prime example, functioning on the analysis of customer behavior and purchase history. This sophisticated algorithm drives about 35% of the company’s total sales, showcasing how data-driven decisions can lead to substantial revenue increases.
- Target: Target effectively employs big data to predict consumer purchasing behavior. By examining shopping data, the company can identify trends and tailor marketing strategies accordingly, even predicting significant life events for customers, leading to personalized marketing efforts.
Industries Benefiting from Big Data
Various sectors are reaping the rewards of big data analytics, which has become instrumental in enhancing operational processes and customer engagement.
- Healthcare: Hospitals and healthcare providers analyze patient data to improve treatment outcomes and operational efficiency. For instance, the Mount Sinai Health System utilizes predictive analytics to forecast patient admissions, allowing for better resource allocation.
- Finance: Financial institutions leverage big data to detect fraud and assess credit risks. JPMorgan Chase uses advanced algorithms to analyze transaction patterns, which aids in identifying suspicious activities and safeguarding assets.
- Retail: Retailers are using big data to optimize supply chain management and inventory control. Walmart, for example, analyzes sales data in real-time to adjust inventory levels and ensure product availability, ultimately enhancing customer satisfaction.
Challenges in Big Data Implementation
Despite the advantages, organizations face several challenges when adopting big data technologies. Understanding these obstacles is crucial for successful integration.
- Data Quality: Ensuring the accuracy and consistency of data is a common challenge. Poor quality data can lead to incorrect insights, hampering decision-making processes.
- Integration: Integrating disparate data sources can be complex. Many organizations struggle to consolidate data from various platforms, which can hinder analytics efforts.
- Data Privacy: With increasing regulations on data privacy, companies must navigate legal frameworks while handling customer data, which can complicate data collection and usage.
“The successful implementation of big data analytics can provide a significant competitive advantage, helping organizations to make informed decisions and drive innovation.”
Future of Big Data and Decision Making
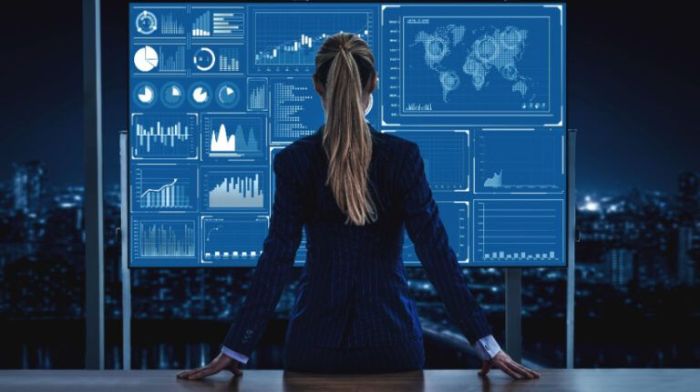
As the landscape of big data continues to evolve, its influence on decision-making processes is expected to grow exponentially in the coming decade. Organizations will increasingly leverage large datasets to enhance their strategic initiatives, drive innovations, and respond to market dynamics in real time. The future of big data isn’t just about volume; it’s about how effectively organizations can interpret and apply insights drawn from complex datasets to make informed decisions.The next wave of big data will be characterized by advancements in artificial intelligence (AI) and machine learning (ML), which will facilitate more sophisticated analyses and predictive capabilities.
Companies will harness these technologies to derive insights that are not only reactive but also proactive, enabling them to anticipate market trends and customer behaviors before they fully materialize. This predictive analysis will be crucial for businesses aiming to maintain a competitive edge in an increasingly data-driven world.
Emerging Trends in Big Data
Several key trends are expected to shape the future of big data and its role in decision-making:
- Real-Time Data Processing: The demand for real-time analytics will grow as organizations seek immediate insights to drive operational efficiency and customer satisfaction. Technologies such as Apache Kafka and Apache Flink will play a pivotal role in enabling real-time data streams.
- Integration of AI and ML: The integration of AI and ML algorithms will enhance the analytical capabilities of big data platforms, allowing for deeper insights and automation of decision-making processes.
- Hybrid Cloud Solutions: Organizations will increasingly adopt hybrid cloud environments to manage big data workloads, balancing the need for scalability and security while optimizing costs.
- Data Democratization: A trend towards making data accessible to all employees, regardless of technical expertise, will empower teams to make data-driven decisions without relying solely on data scientists.
- Increased Focus on Data Quality: As organizations collect more data, ensuring its accuracy, relevance, and timeliness will be essential for effective decision-making.
Ethical Considerations and Risks
While the potential of big data is immense, ethical considerations and risks must be navigated carefully. Key areas of concern include:
- Data Privacy: With increasing regulations such as GDPR and CCPA, organizations must prioritize data privacy and establish clear policies to protect user information.
- Bias in Data: The presence of biased data can lead to skewed analytics, resulting in unfair or discriminatory decision-making outcomes. Companies must adopt methodologies to identify and mitigate biases in their datasets.
- Security Risks: The accumulation of vast amounts of sensitive data poses significant security challenges. Organizations must invest in robust cybersecurity measures to protect against data breaches.
- Transparency: Transparency in data usage and algorithmic processes is vital for building trust with customers and stakeholders. Companies should disclose how data is collected, analyzed, and applied in decision-making.
- Ethical AI Use: The deployment of AI in decision-making raises ethical questions about accountability and the potential for misuse. Organizations should establish ethical guidelines governing the use of AI and machine learning.
Framework for Future Adaptation
To navigate the evolving landscape of big data, organizations can adopt a strategic framework that addresses upcoming challenges:
- Develop a Data Governance Strategy: Establish clear policies related to data management, usage, and security to ensure compliance and ethical practices.
- Invest in Employee Training: Equip employees with the necessary skills to interpret data effectively and utilize big data tools to enhance decision-making capabilities.
- Focus on Collaboration: Encourage interdepartmental collaboration to create a culture of data sharing and collective decision-making, leveraging diverse insights across the organization.
- Embrace Agile Methodologies: Implement agile approaches to adapt swiftly to changing data landscapes and emerging technologies.
- Monitor Emerging Technologies: Stay informed about advancements in data analytics and AI, ensuring the organization remains competitive and capable of leveraging new tools efficiently.