How to analyze data for business intelligence decisions takes center stage in today’s data-driven landscape, where organizations strive to harness the power of information for strategic advantage. The ability to collect, clean, visualize, and analyze data is crucial for making informed decisions that propel businesses forward. By understanding the various methodologies and tools available, companies can transform raw data into actionable insights that foster growth and innovation.
This exploration begins with data collection techniques, highlighting effective methods and tools to gather vital information. It emphasizes the significance of data quality through cleaning and preparation processes, ensuring reliability in analysis. The discussion further delves into visualization strategies, showcasing how presenting data effectively can influence decision-making, followed by the analytical techniques that guide businesses in crafting strategic choices based on empirical evidence.
Finally, the implementation of robust business intelligence systems is examined, underscoring the importance of user training and monitoring key performance indicators for sustained success.
Data Collection Techniques
Data collection is a crucial aspect of business intelligence, providing the foundation for informed decision-making. Effective data gathering allows organizations to derive insights, track performance, and strategize for future growth. Understanding the various methods of data collection and using the right tools can significantly enhance the quality of analysis.Various methods to gather information for business intelligence include surveys, interviews, observations, and secondary data analysis.
Each method has its unique strengths and is suited for different types of data requirements. Selecting the appropriate technique is paramount in ensuring the reliability and relevance of the collected data.
Survey Design for Data Gathering
Crafting effective surveys is essential for collecting meaningful data. A well-designed survey can yield actionable insights, while a poorly structured one may result in misleading conclusions. Key considerations for designing surveys include clarity of questions, response options, and the overall structure of the survey. To enhance the effectiveness of surveys, consider the following elements:
- Clear Objectives: Define what information you aim to gather and ensure questions align with these objectives.
- Question Types: Utilize a mix of open-ended, closed-ended, and rating scale questions to capture diverse data.
- Pilot Testing: Conduct a pilot test with a small audience to identify any ambiguity in questions and improve clarity.
- Length and Timing: Keep the survey concise to maintain engagement and avoid survey fatigue among respondents.
Tools for Data Collection
Numerous tools are available for data collection, each offering unique features and advantages that cater to diverse needs. The selection of tools depends on factors such as the target audience, data type, and the desired analysis.Some popular data collection tools include:
- SurveyMonkey: This platform allows users to create surveys with a user-friendly interface, offering various templates and question types.
- Google Forms: A free tool that simplifies survey creation and data collection, enabling real-time collaboration and easy data export to Google Sheets.
- Qualtrics: A robust survey tool with advanced analytics capabilities, ideal for enterprises looking for detailed insights.
- Tableau: While primarily a data visualization tool, Tableau also facilitates data gathering through integration with other data sources and platforms.
The benefits of using these tools include streamlined data collection processes, enhanced data accuracy, and improved user experiences. By leveraging technology, businesses can ensure that data collection is efficient, effective, and aligned with their strategic goals.
Implementing business intelligence effectively requires an understanding of best practices for business intelligence implementation. This ensures that organizations can harness data-driven insights efficiently. By aligning BI strategies with company goals and fostering a culture of data literacy, businesses can maximize their analytical potential and drive better decision-making across all levels.
“The quality of your decisions is only as good as the quality of your data.”
In today’s data-driven landscape, recognizing the importance of data visualization in business intelligence is crucial. Effective visualization helps stakeholders grasp complex data trends quickly, allowing for timely and informed decisions. By presenting data in a visually appealing manner, businesses can enhance comprehension and engagement, ultimately leading to more actionable insights.
Data Cleaning and Preparation: How To Analyze Data For Business Intelligence Decisions
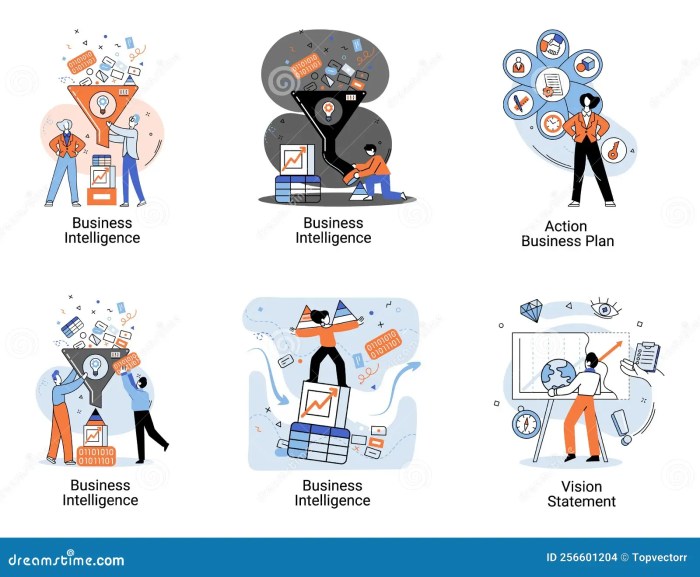
Data cleaning and preparation are pivotal steps in the data analysis process, particularly in the context of business intelligence. Data quality directly influences the reliability of insights derived from analytics. Poor data quality can lead to misguided decisions, affecting an organization’s performance and strategy. Hence, it is essential to invest time and resources into ensuring that data is accurate, consistent, and up-to-date before conducting any analysis.The process of preparing data typically involves several systematic steps that enhance its quality and usability.
These steps include transformation and normalization, which are crucial for making data ready for analysis. During transformation, raw data is converted into a format that is more suitable for analytical purposes. Normalization, on the other hand, ensures that the data follows a consistent scale, which is vital for comparative analysis.
Importance of Data Quality and Cleaning Processes
High-quality data is indispensable for effective business intelligence. The cleaning process eliminates inaccuracies and inconsistencies, ensuring that the analysis yields valid results. This step is especially critical when dealing with large datasets, where errors can significantly skew findings. Key steps in data cleaning and preparation include:
- Identifying Data Types: Understanding the different types of data (e.g., categorical, numerical) helps in selecting the appropriate cleaning techniques.
- Data Transformation: This includes converting data into a suitable format, such as changing date formats or categorizing numerical ranges.
- Normalization: Adjusting values to a common scale is crucial for accurate analysis, particularly with metrics that may vary widely.
Handling Missing or Inconsistent Data
Dealing with missing or inconsistent data is a common challenge in data preparation. Such issues can arise due to various reasons, such as data entry errors or system malfunctions. Addressing these inconsistencies is vital to maintain the integrity of the analysis.Methods to handle missing data include:
- Imputation: Replacing missing values with estimated ones, such as mean, median, or mode, based on available data.
- Deletion: Removing records with missing data, applicable when the volume of missing information is minimal and does not significantly affect overall data quality.
- Flagging: Keeping track of missing data by marking it, which allows for further analysis on its impact on results.
Inconsistent data can also be addressed through validation rules and standardization practices. For instance, ensuring all entries follow a consistent naming convention or format enhances overall data quality and facilitates easier analysis. By rigorously cleaning and preparing data, businesses can significantly enhance the accuracy of their insights, leading to better decision-making.
Data Visualization Strategies
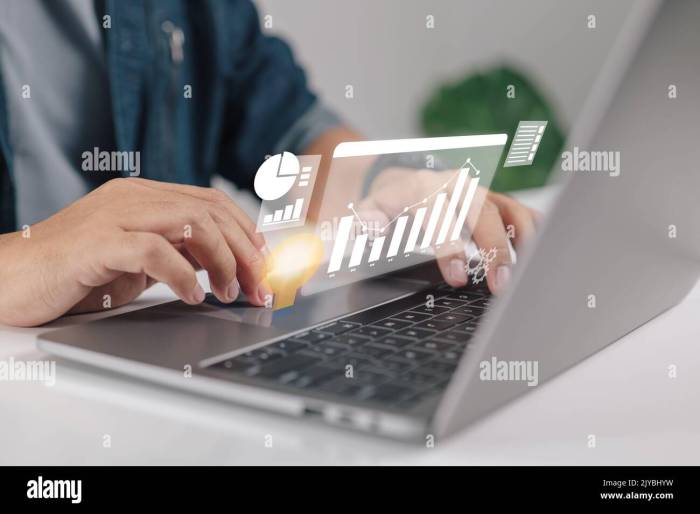
Data visualization plays a crucial role in transforming complex data sets into comprehensible visual formats that facilitate decision-making in business intelligence. By employing effective visualization techniques, organizations can uncover trends, patterns, and insights that might otherwise remain hidden in raw data. This section discusses various visualization techniques, the significance of dashboards, and evaluates software options for effective data representation.
Visualization Techniques for Business Intelligence Data
Utilizing appropriate visualization techniques is essential for presenting data effectively. Each method can reveal different aspects of the data and cater to various audience needs. The following list Artikels key visualization techniques that enhance data interpretation:
- Bar Charts: Ideal for comparing quantities across different categories, bar charts provide a clear visual representation of data differences.
- Line Graphs: Useful for displaying trends over time, line graphs help to visualize changes and patterns in data points across a continuous scale.
- Pie Charts: Effective for illustrating proportions, pie charts help in understanding the relative sizes of components within a whole.
- Heat Maps: Emphasizing the intensity of data values, heat maps use color gradations to represent data density and distribution, providing immediate visual cues.
- Scatter Plots: These are effective for showing relationships between two variables, allowing viewers to discern correlations or clusters within the data.
- Infographics: Combining graphics with data patterns, infographics are useful for storytelling, making complex data accessible and engaging for a broader audience.
Dashboards and Their Components in Data Representation
Dashboards serve as a central hub for monitoring key performance indicators (KPIs) and overall business performance. They provide a consolidated view of critical data points, enabling decision-makers to assess performance at a glance. Key components of an effective dashboard include:
- Data Widgets: These are interactive elements that display visualizations, such as graphs, charts, and tables, allowing users to engage with the data.
- Filters: Filters enable users to customize the data view based on specific parameters, such as date ranges or categories, enhancing user experience and relevance.
- Alerts and Notifications: Dashboards may include features that notify users of significant changes or thresholds, ensuring timely responses to critical developments.
- Real-Time Data Integration: Effective dashboards incorporate real-time data updates, enabling users to make informed decisions based on the most current information.
- Mobile Compatibility: With the increasing need for on-the-go access, dashboards that are mobile-friendly provide flexibility and extend usability beyond traditional desktop environments.
Software Options for Effective Data Visualization, How to analyze data for business intelligence decisions
Choosing the right software for data visualization is essential for maximizing the effectiveness of visual strategies. Various options are available, each offering unique features that cater to different business needs. Below is a comparison of popular data visualization software:
Software | Features | Ideal Use Case |
---|---|---|
Tableau | Drag-and-drop interface, extensive data connectors, interactive dashboards | Suitable for large datasets requiring complex visual analysis |
Microsoft Power BI | Integration with other Microsoft tools, customizable reports, real-time dashboard updates | Best for organizations already using Microsoft products |
QlikView | Associative data indexing, user-friendly interface, advanced analytics | Ideal for businesses needing interactive and intuitive data discovery |
Looker | Data modeling capabilities, embedded analytics, real-time data exploration | Effective for companies focused on leveraging data for strategic insights |
D3.js | Customizable visualizations, powerful data-driven documents, web-based | Best suited for developers looking for highly customized visualization solutions |
Analytical Techniques for Decision-Making
In today’s data-driven business landscape, the ability to leverage analytical techniques is crucial for informed decision-making. Businesses utilize various analytical methods to uncover insights from data, enabling them to forecast trends, understand consumer behavior, and optimize operations. This segment delves into the different analytical techniques applicable in business contexts, Artikels how to construct a decision-making framework with these tools, and showcases real-world examples where data-driven decisions have led to successful outcomes.
Predictive Analytics and Trend Analysis
Predictive analytics involves using historical data, statistical algorithms, and machine learning techniques to identify the likelihood of future outcomes based on past behavior. This approach helps businesses anticipate market trends, customer preferences, and operational challenges. Trend analysis, on the other hand, focuses on observing historical data over a specified period to identify consistent results or patterns. Both techniques are instrumental in forming a robust decision-making framework that can adapt to changing market dynamics.
Here are several key points regarding predictive analytics and trend analysis:
- Forecasting Sales: Companies can utilize predictive analytics to forecast future sales based on historical sales data, seasonality, and market trends, allowing for better inventory management.
- Customer Behavior Insights: By analyzing past purchasing behaviors, businesses can predict future buying patterns and tailor marketing strategies accordingly, enhancing customer engagement.
- Risk Management: Predictive models help organizations assess potential risks by evaluating past incidents, enabling proactive measures to mitigate future issues.
- Operational Efficiency: Trend analysis can reveal inefficiencies in workflows, prompting organizations to optimize processes and reduce costs.
“Predictive analytics transforms data into actionable insights, enabling organizations to anticipate market changes and respond proactively.”
Designing a Decision-Making Framework
A decision-making framework integrates analytical tools and methodologies to guide business leaders in making informed choices. When designing this framework, several components should be considered to ensure efficacy and relevance:
- Define Objectives: Clearly Artikel what the organization hopes to achieve through data analysis, such as increasing revenue or improving customer satisfaction.
- Select Appropriate Tools: Choose analytical tools that align with business objectives, whether they are statistical software, visualization tools, or machine learning platforms.
- Establish Data Governance: Implement policies for data quality, security, and accessibility to maintain the integrity of the analysis.
- Iterate and Adapt: Continuously refine the framework based on feedback and changing business landscapes, updating analytical methods and goals as necessary.
“A structured decision-making framework ensures that analytical insights are effectively translated into actionable business strategies.”
Case Studies of Data-Driven Decisions
Real-world case studies illustrate the power of data-driven decision-making in achieving organizational success. One notable example is Netflix, which employs predictive analytics to inform content creation and recommendation systems. By analyzing viewer preferences and behaviors, Netflix can not only predict which genres will be popular but also decide on new content to invest in, thus increasing user satisfaction and retention.Another compelling case is Target, which successfully utilized data analytics to predict consumer purchasing behavior and improve marketing strategies.
By analyzing shopping patterns, Target was able to identify key products that attracted pregnancy-related purchases, allowing them to create targeted marketing campaigns that significantly boosted sales.These examples demonstrate how companies can harness analytical techniques to drive strategic decisions, optimize resources, and enhance customer experiences, ultimately leading to improved business performance.
Implementation of Business Intelligence Systems
The implementation of a Business Intelligence (BI) system is a critical venture for organizations aiming to enhance data-driven decision-making. This process requires careful planning, execution, and ongoing management to realize the full potential of BI tools and methodologies. Understanding the necessary steps and considerations can significantly increase the likelihood of a successful implementation.
Steps for Implementing a Business Intelligence System
To successfully implement a BI system, organizations should follow a structured approach. The following steps Artikel the essential phases of this process:
- Define Objectives and Requirements: Clearly articulate the specific goals of the BI system, identifying what data insights are needed to improve decision-making processes.
- Assess Current Infrastructure: Evaluate the existing IT infrastructure to determine compatibility with the chosen BI tools and identify necessary upgrades or changes.
- Select BI Tools and Technologies: Choose the appropriate BI software and technologies that align with the organization’s goals, ensuring they integrate well with existing systems.
- Data Integration: Gather data from various sources and consolidate it into a centralized repository, ensuring that it is accessible and analyzable.
- Data Quality Assurance: Implement data cleaning and validation processes to ensure accuracy, consistency, and reliability of the data used in the BI system.
- User Training and Support: Provide comprehensive training for end-users to ensure they can effectively utilize the BI tools, along with ongoing support for troubleshooting and enhancements.
- Monitor and Evaluate: Continuously assess the BI system’s performance against predefined KPIs, making adjustments to optimize its effectiveness.
Key Performance Indicators for Effective Decision-Making
Monitoring specific Key Performance Indicators (KPIs) is crucial for assessing the impact of BI systems on business outcomes. KPIs should align with organizational objectives and help measure the effectiveness of decision-making processes. Key KPIs to consider include:
- Revenue Growth: Tracks the increase in revenue attributable to data-driven decisions made using BI insights.
- Customer Satisfaction Scores: Measures the impact of BI on enhancing customer experiences, reflecting the success of targeted initiatives.
- Operational Efficiency: Assesses improvements in processes and resource management as a direct result of BI analysis.
- Time to Insight: Evaluates the speed at which data is transformed into actionable insights, impacting responsiveness and agility.
- Return on Investment (ROI): Calculates the financial return from BI investments, comparing costs against benefits realized through improved decision-making.
Importance of User Training and Support
Successful adoption of a BI system is heavily reliant on user training and ongoing support. Adequate training empowers users to leverage the full functionality of BI tools, thereby maximizing their contributions to data-driven decision-making. The importance of this aspect can be highlighted through the following considerations:
“User engagement in BI systems is paramount; without proper training, even the most sophisticated tools can go underutilized.”
Organizations must invest in structured training programs that cater to different user roles, ensuring that all employees have the necessary skills to operate the BI system effectively. Furthermore, establishing a support framework can facilitate continuous learning and problem-solving, fostering a data-centric culture where users feel confident in their ability to make informed decisions based on data insights.