How to ensure data quality in business intelligence is a pivotal topic that resonates across every sector. In an age where data drives decision-making, the integrity of that data determines the success or failure of business initiatives. Poor data quality can lead to flawed insights, resulting in misguided strategies and financial losses. Understanding the significance of data quality, the common pitfalls, and how to avoid them is essential for organizations seeking to thrive in a competitive landscape.
With a clear framework in mind, businesses can adopt best practices to maintain high data standards, implement robust validation processes, and conduct regular audits. This ensures that the information at hand is not only accurate but also actionable, enabling informed decisions that propel growth and innovation.
Importance of Data Quality in Business Intelligence
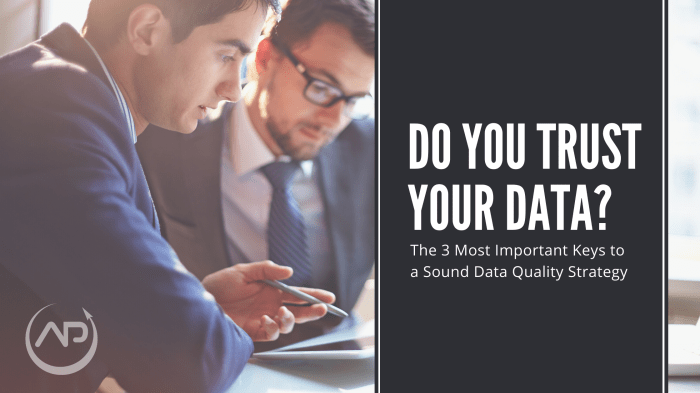
In the realm of business intelligence (BI), data quality plays a critical role in shaping insightful decision-making processes. Organizations rely heavily on accurate, timely, and relevant data to inform their strategies and optimize operations. Poor data quality can lead to misguided decisions, wasted resources, and missed opportunities, ultimately undermining competitive advantages and operational efficiency.The impact of data quality on decision-making cannot be overstated.
High-quality data provides a solid foundation for analysis, enabling businesses to identify trends, forecast outcomes, and make informed decisions. Conversely, poor data quality can lead to erroneous conclusions and misguided strategies. For example, if a company bases its marketing strategy on faulty customer data, it may target the wrong audience, resulting in ineffective campaigns and loss of revenue.
Financial Implications of Poor Data Quality
The financial implications of poor data quality can be severe and far-reaching. Organizations may experience significant costs associated with rectifying data errors and inaccuracies. Moreover, these costs extend beyond administrative expenses. The following points illustrate the financial impact:
- Increased operational costs: Organizations often spend considerable resources on data cleaning and validation processes to rectify inaccuracies.
- Loss of revenue: Inaccurate data can lead to poor market strategies, resulting in lost sales opportunities and diminished customer trust.
- Regulatory fines: In industries that are heavily regulated, non-compliance due to poor data quality can lead to substantial fines and legal repercussions.
The cumulative effect of these financial implications can be staggering. A study by IBM estimated that poor data quality costs the U.S. economy approximately $3.1 trillion annually. This staggering figure underscores the importance of maintaining high data quality standards in business intelligence initiatives.
Common Data Quality Issues in Business Intelligence
Common data quality issues that affect business intelligence are multifaceted, often stemming from inadequate data management practices. Identifying and addressing these issues is essential for ensuring reliable data-driven insights. The following list Artikels prevalent data quality challenges:
- Data duplication: Duplicate records can lead to inflated metrics, skewed analyses, and wasted resources.
- Inconsistent data formats: Variability in data formatting can hinder integration efforts and complicate data analysis.
- Incomplete data: Missing information can lead to inaccurate conclusions and diminished confidence in reports.
- Outdated information: Relying on obsolete data can result in misguided strategies and poor decision-making.
Recognizing these common data quality issues and implementing effective data governance practices can enhance the quality of insights derived from business intelligence. By prioritizing data quality, organizations position themselves for more informed decision-making and improved operational outcomes.
Choosing the right business intelligence tools is crucial for any organization looking to leverage data effectively. To make an informed decision, it’s essential to assess your specific needs and understand the features offered. For detailed guidance, check out this insightful article on how to choose business intelligence tools effectively.
Best Practices for Ensuring Data Quality

Data quality is paramount in business intelligence, as it directly impacts decision-making processes and organizational success. Implementing best practices can significantly enhance the reliability and usability of data throughout its lifecycle. By adhering to standardized techniques, organizations can ensure that their data remains accurate, consistent, and relevant.
Standard Practices to Maintain Data Quality, How to ensure data quality in business intelligence
To maintain high data quality, organizations should incorporate a set of standard practices that are consistently applied across all data management processes. These practices not only improve data integrity but also streamline operations and enhance reporting accuracy. The following list Artikels essential practices for ensuring data quality:
- Implement Data Governance Frameworks: Establish clear policies and procedures for data management, including ownership and accountability structures.
- Standardize Data Entry: Develop standardized formats for data entry to reduce errors caused by inconsistent inputs.
- Train Staff on Data Management: Provide regular training sessions for employees on best practices in data handling and quality assurance.
- Utilize Data Profiling Tools: Employ tools to assess the quality of data, identifying anomalies and areas for improvement.
- Regularly Update Data: Ensure that data is periodically reviewed and updated to reflect the most current and relevant information.
Methods for Implementing Data Validation Checks
Data validation checks are critical to ensuring that the data collected and used in business intelligence is accurate and reliable. These checks enable organizations to identify and rectify errors before they impact decision-making. Effective methods for implementing data validation checks include:
- Define Validation Rules: Establish specific criteria that data must meet before being accepted into the system, such as format checks and range checks.
- Automate Validation Processes: Utilize software solutions that automatically validate data upon entry, minimizing human error.
- Conduct Manual Review: Supplement automated checks with periodic manual reviews of data to catch errors that automated systems may overlook.
- Implement Error Reporting Mechanisms: Create systems for reporting and addressing data errors swiftly to maintain data integrity.
Step-by-Step Procedure for Regular Data Audits
Conducting regular data audits is an essential practice for maintaining data quality. A systematic approach ensures that any issues are identified and addressed promptly, thereby preserving the integrity of business intelligence processes. The following step-by-step procedure Artikels how to effectively perform data audits:
- Define Audit Scope: Determine the specific datasets and processes to be audited, ensuring relevance to business objectives.
- Gather Data: Collect the necessary data from various sources, ensuring it is comprehensive and representative.
- Analyze Data Quality: Evaluate the data against established quality metrics, assessing accuracy, completeness, consistency, and timeliness.
- Document Findings: Record any discrepancies or issues identified during the audit, noting their potential impact on business operations.
- Develop Action Plans: Create actionable plans to address identified issues, assigning responsibilities and deadlines for resolution.
- Implement Changes: Execute the action plans, making necessary adjustments to processes and systems to enhance data quality.
- Review and Follow-Up: Establish a timeline for reviewing the effectiveness of the changes made, ensuring continuous improvement in data quality.
Tools and Technologies for Data Quality Management
Ensuring data quality is paramount in the realm of business intelligence. With the increasing reliance on data-driven decision-making, organizations need robust tools and technologies to effectively manage their data quality. These tools assist in data cleansing, monitoring, and governance, providing businesses with reliable, accurate, and actionable insights.The landscape of data quality management is rich with innovative solutions that enhance the integrity and usability of data.
Automation plays a critical role as it not only streamlines processes but also reduces human error, ensuring that the data remains consistent and reliable. Below are some prominent categories of tools and technologies that contribute significantly to data quality management.
Implementing business intelligence requires strategic planning to ensure success. Organizations should adhere to best practices for business intelligence implementation , which include defining clear objectives, ensuring data quality, and engaging stakeholders throughout the process. These steps can significantly enhance the effectiveness of your BI initiatives.
Popular Tools for Data Cleansing and Monitoring
Various tools are available in the market that cater to data cleansing and monitoring needs. These tools can automate the process of identifying and correcting errors in datasets. The following list highlights a few noteworthy tools, along with their key features:
- Talend Data Quality: An open-source tool that provides data profiling, cleansing, and enrichment capabilities. It offers a user-friendly interface and integrates seamlessly with other Talend products.
- Informatica Data Quality: A powerful tool that delivers comprehensive data quality management through profiling, cleansing, and monitoring. It supports multiple data sources and provides a robust set of functionalities for data governance.
- Microsoft Azure Data Factory: This cloud-based tool facilitates data integration and transformation, ensuring that data quality is maintained throughout the ETL (Extract, Transform, Load) process.
- Trifacta: Known for its data wrangling capabilities, Trifacta provides data preparation solutions that enhance data quality by allowing users to visualize and clean their datasets efficiently.
The selection of the appropriate tool often depends on the specific requirements of a business, including the complexity of data, the volume of information being processed, and the existing technology stack.
Role of Automation in Enhancing Data Quality
Automation has become a cornerstone in the pursuit of high-quality data. By automating repetitive tasks, organizations can minimize the potential for human error and accelerate their data management processes. Automation in data quality management can encompass several aspects:
- Data Profiling: Automated profiling tools can analyze data sets and provide insights into data quality metrics, such as completeness, consistency, and accuracy.
- Data Cleansing: Automated cleansing processes can efficiently address duplicates, missing values, and formatting issues, ensuring data is ready for analysis.
- Data Monitoring: Continuous monitoring solutions can automatically flag anomalies or discrepancies in data, allowing organizations to address issues in real-time.
- Integration with Machine Learning: Advanced automation tools leverage machine learning algorithms to predict data quality issues and suggest corrective actions, leading to improved data accuracy over time.
By implementing automation, businesses can not only save time and resources but also achieve a higher level of confidence in their data, which is critical for informed decision-making.
Comparison of Software Solutions Based on Features
When evaluating software solutions for data quality management, it is essential to consider various features that can impact the effectiveness of these tools. Below is a comparative overview of key features offered by popular data quality management tools:
Tool | Data Profiling | Data Cleansing | Real-time Monitoring | Integration Capability |
---|---|---|---|---|
Talend Data Quality | Yes | Yes | Yes | High |
Informatica Data Quality | Yes | Yes | Yes | Very High |
Microsoft Azure Data Factory | Limited | Yes | Yes | High |
Trifacta | Yes | Yes | Limited | Moderate |
This comparison illustrates how different tools cater to the diverse needs of organizations. By carefully considering the features and functionalities of each tool, businesses can select the right solution to enhance their data quality management strategies effectively.
Training and Culture for Data Quality: How To Ensure Data Quality In Business Intelligence
Establishing a robust framework for data quality in business intelligence necessitates an emphasis on training employees and nurturing a culture that values data integrity. Ensuring that all staff members understand the significance of data quality is fundamental to an organization’s success. It shapes how data is collected, processed, and utilized across all levels of the business.Fostering a data-centric culture requires intentional strategies that engage employees at every level, aligning their roles with the organization’s data quality goals.
This culture is built not just on policies, but on the ongoing education and support of the workforce, creating champions for data quality throughout the organization.
Importance of Employee Training on Data Quality
Training employees on data quality is essential because it equips them with the necessary skills and knowledge to handle data responsibly and efficiently. Proper training ensures that employees understand the standards and procedures for data entry, management, and analysis. This includes familiarity with data governance principles, compliance standards, and best practices for data handling. Moreover, well-trained employees are more likely to identify inconsistencies and errors in data early on, which mitigates risks and enhances the reliability of the insights derived from data analysis.
An organization that prioritizes data quality training essentially invests in its own success by empowering its workforce.
Designing a Program for a Data-Centric Culture
To cultivate a data-centric culture, organizations should implement a comprehensive training program that includes the following elements:
- Onboarding Training: New employees should receive training on data quality standards as part of their onboarding process. This establishes a baseline understanding from day one.
- Ongoing Workshops: Regular workshops and seminars on data management techniques and updates in data governance policies keep employees informed and engaged.
- Certification Programs: Providing opportunities for employees to earn certifications in data management can motivate them to deepen their knowledge and skills.
- Cross-Department Collaborations: Encourage collaboration between departments to foster a holistic understanding of how data quality impacts various business functions.
The focus of this program should be on creating a shared responsibility for data quality among all employees, emphasizing that everyone plays a role in maintaining data integrity.
Strategies for Promoting Continuous Improvement in Data Handling
Continuous improvement in data handling practices can be promoted through various strategies that encourage feedback and adaptation to changing data environments. These strategies ensure that the organization remains proactive rather than reactive in its approach to data quality.
- Establish Feedback Loops: Regularly seek feedback from employees regarding data handling processes. Use surveys and focus groups to gather insights on challenges they face, which can inform improvements.
- Performance Metrics: Implement performance metrics to evaluate data quality initiatives. Metrics like accuracy rates, error rates, and turnaround times can help identify areas needing improvement.
- Incentivizing Quality Contributions: Recognize and reward employees and teams that consistently contribute to data quality improvements. This can motivate others to prioritize data integrity in their daily tasks.
- Continuous Learning Opportunities: Encourage a culture of continuous learning by offering access to online courses, conferences, or literature that keeps employees informed about the latest trends and technologies in data management.
By integrating these strategies into the organizational framework, companies can continuously enhance their data handling capabilities, leading to improved decision-making and operational efficiency.
Case Studies and Real-World Applications
In the realm of business intelligence, data quality is not merely a technical concern but a crucial driver of organizational performance. Numerous businesses have successfully leveraged data quality initiatives to enhance their operational efficiency and decision-making processes. By examining real-world cases, we can gain insights into the tangible benefits of effective data quality management and the lessons learned from various challenges.
Successful Data Quality Management Examples
Several organizations have implemented robust data quality initiatives that resulted in measurable improvements in their business performance. The following examples illustrate these successful transformations:
- Procter & Gamble: This multinational consumer goods company undertook a comprehensive data quality project that improved their supply chain management. By enhancing data accuracy and consistency across multiple systems, P&G achieved a 15% reduction in inventory costs, streamlining operations and enhancing customer satisfaction.
- American Express: Faced with the challenge of managing vast amounts of customer data, American Express implemented data governance policies to address inconsistencies and inaccuracies. As a result, the company improved customer insights, leading to a 20% increase in targeted marketing campaign effectiveness.
- Walmart: By focusing on data integrity, Walmart enhanced its inventory management system. The initiative reduced stockouts and overstocks, yielding an impressive increase in sales by 10% due to better product availability and customer experience.
Lessons Learned from Data Quality Challenges
Organizations often encounter significant challenges in maintaining high standards of data quality. The following case studies highlight the lessons learned from these difficulties:
- Target: After a failed data integration project, Target faced significant backlash due to poor data quality relating to customer preferences. The company learned the importance of establishing a robust data governance framework to ensure data accuracy and reliability before any large-scale analysis or application.
- Equifax: The data breach incident at Equifax highlighted severe vulnerabilities related to data quality and security. The organization recognized the necessity for continuous monitoring and auditing of data quality metrics, which led to an overhaul of their data management practices to prevent future incidents.
- Volkswagen: Volkswagen encountered data quality issues that contributed to inconsistencies in emissions reporting. This experience underscored the need for transparency and accountability in data management, prompting the company to adopt stricter protocols and validation processes to maintain data integrity.
Successful data quality initiatives not only enhance decision-making but also significantly impact overall business performance, as illustrated by these compelling case studies.