How to leverage big data in business intelligence opens the door to the future of informed decision-making and unparalleled competitive edge. In today’s fast-paced business landscape, harnessing the power of big data is crucial for organizations aiming to thrive. By integrating vast amounts of information from diverse sources, companies can not only enhance their decision-making processes but also uncover invaluable insights that drive innovation and growth.
From understanding consumer behavior to optimizing operations, big data plays a pivotal role in shaping strategies across various industries. The ability to process real-time data allows businesses to respond swiftly to market changes, ensuring they remain one step ahead of competitors. As we delve deeper into the tools, techniques, and success stories surrounding big data, it’s clear that the potential for transformative impact is immense.
Importance of Big Data in Business Intelligence: How To Leverage Big Data In Business Intelligence
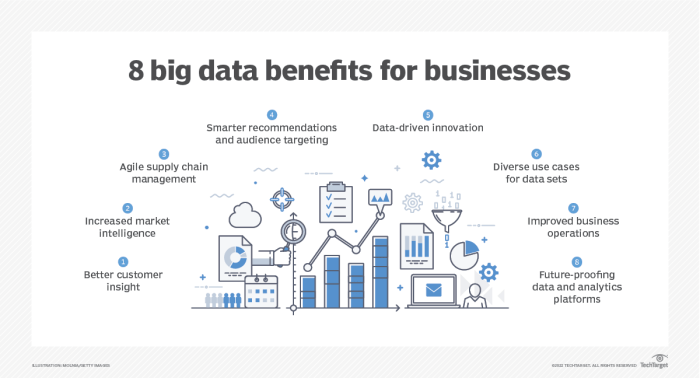
Big data plays a crucial role in modern business intelligence, acting as the backbone for informed decision-making and strategic planning. In an era where data is generated at unprecedented rates, leveraging big data enables organizations to glean insights that drive efficiency, innovation, and profitability. By harnessing vast quantities of information, businesses can uncover patterns and trends that significantly enhance operational effectiveness.Big data enhances decision-making processes by providing real-time insights into customer behavior, market trends, and operational performance.
Ensuring data quality is crucial for effective business intelligence. Companies can achieve this by implementing stringent data validation processes and regular audits, which are essential steps outlined in how to ensure data quality in business intelligence. By focusing on these strategies, organizations can enhance decision-making and drive better outcomes.
This capability allows businesses to make data-driven decisions rather than relying on gut feelings or outdated information. For instance, retailers utilize big data analytics to optimize inventory management and personalize marketing strategies, leading to better customer satisfaction and increased sales.
Competitive Advantage through Big Data
The ability to harness big data effectively drives competitive advantage across various industries. Companies that capitalize on analytics gain insights that not only improve their operations but also enhance their market positioning. Significant areas include:
- Healthcare: Big data analytics enables healthcare providers to predict patient outcomes and improve treatment protocols, resulting in better patient care and reduced costs.
- Finance: Financial institutions use big data to detect fraudulent activities, assess risks more accurately, and personalize service offerings for clients, thus maintaining an edge in the competitive market.
- Manufacturing: Data analytics helps manufacturers implement predictive maintenance, thereby reducing downtime and optimizing production processes, which leads to cost savings and improved product quality.
- Retail: Retailers analyze customer data to tailor promotional strategies, manage supply chains more efficiently, and enhance customer experiences, directly impacting their sales growth.
The integration of big data into the business model empowers firms to anticipate market fluctuations and consumer preferences, ensuring they remain relevant and competitive in their respective fields.
Real-Time Data Processing in Business Intelligence
Real-time data processing is essential for business intelligence as it allows companies to react swiftly to changing conditions. The significance of real-time analytics cannot be overstated; it transforms the way businesses operate by enabling immediate responses to challenges and opportunities. Key aspects of real-time data processing include:
- Instant Decision Making: Access to real-time data empowers organizations to make informed decisions on the spot, minimizing potential losses and maximizing opportunities.
- Customer Engagement: Businesses can engage with customers in real-time, providing personalized experiences and improving customer satisfaction through immediate feedback and support.
- Operational Efficiency: Real-time insights help streamline operations, allowing businesses to monitor performance metrics continuously and adapt strategies as needed.
- Risk Management: Organizations can identify and mitigate risks as they arise, utilizing predictive analytics to foresee potential issues before they escalate.
Implementing real-time data processing not only enhances responsiveness but also fosters a culture of agility and innovation, positioning businesses for long-term success in a data-driven landscape.
For small businesses, staying ahead in the competitive landscape requires adapting to emerging technologies. One of the key areas to watch are the trends in business intelligence for small businesses , which highlight innovative tools and techniques that can optimize operations and offer valuable insights. Embracing these trends can propel growth and efficiency.
Tools and Technologies for Leveraging Big Data
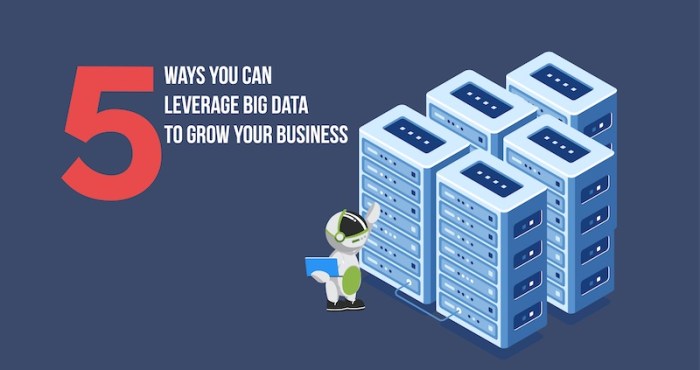
The landscape of business intelligence is rapidly evolving with the rise of big data. To fully harness the power of this vast resource, organizations must utilize a variety of tools and technologies that enable them to analyze, store, and process data effectively. Understanding these tools not only boosts analytical capabilities but also enhances decision-making processes across the board.
Popular Data Analytics Tools for Business Intelligence
A range of data analytics tools have emerged as essential for businesses looking to leverage big data. These tools provide features that facilitate data visualization, predictive analytics, and real-time processing. Their capabilities are crucial for transforming raw data into actionable insights.
- Tableau: Renowned for its robust data visualization capabilities, Tableau allows users to create visually appealing dashboards and reports that simplify complex data insights.
- Microsoft Power BI: A powerful analytics tool that integrates seamlessly with various data sources, Power BI empowers users to share insights and collaborate effectively.
- Apache Hadoop: An open-source framework that supports the distributed processing of large data sets across clusters of computers. Hadoop is essential for managing big data storage and analytics.
- SAS: Known for advanced analytics, SAS offers powerful data management and predictive analytics capabilities, making it a favorite among data professionals.
- QlikView: This tool enables users to visualize data in real-time and supports data discovery, making it easier to uncover insights hidden within large data sets.
Technologies Supporting Big Data Storage and Processing, How to leverage big data in business intelligence
To effectively manage big data, various technologies are utilized that facilitate both storage and processing. These technologies are essential for ensuring that organizations can collect and analyze data without facing performance bottlenecks.
- Apache Kafka: A distributed streaming platform that allows for the real-time processing of data feeds, Kafka is invaluable in scenarios requiring immediate data analysis.
- Amazon S3: A scalable storage service that allows for the storage of any amount of data, S3 is widely used for big data analytics, providing durability and low-latency access.
- NoSQL Databases: Technologies like MongoDB and Cassandra are designed to handle unstructured data, allowing for flexible schema design and horizontal scaling, which is crucial for big data applications.
- Hadoop Distributed File System (HDFS): This file system allows for the storage of large files across multiple machines, providing high throughput access to application data.
- Spark: A powerful open-source processing engine that supports batch and stream processing, Spark is known for its speed and ease of use compared to Hadoop’s MapReduce.
Comparison of Cloud Versus On-Premises Solutions for Big Data Management
When considering solutions for big data management, organizations often grapple with the choice between cloud-based and on-premises systems. Each option presents distinct advantages and challenges that can impact performance, cost, and scalability.
Feature | Cloud Solutions | On-Premises Solutions |
---|---|---|
Scalability | Highly scalable; resources can be adjusted on-demand. | Limited by physical hardware; scaling requires significant investment. |
Cost | Generally lower upfront costs, pay-as-you-go model. | Higher initial investment; ongoing maintenance costs can be substantial. |
Control | Less control over data security and compliance. | More control over data security; compliance can be managed internally. |
Performance | Dependent on internet connectivity; may experience latency. | Generally provides faster access due to local hardware proximity. |
Updates and Maintenance | Automatic updates and maintenance managed by the vendor. | Requires in-house IT resources for updates and maintenance. |
Techniques for Data Integration and Management
In the landscape of big data and business intelligence, integrating diverse data sources effectively is paramount for organizations seeking actionable insights. The ability to unify various data streams into a coherent strategy not only enhances analytical capabilities but also drives informed decision-making. As businesses evolve, the complexity of data sources increases, necessitating robust methods for integration, ensuring data quality and governance, and maintaining security and compliance.
Methods for Integrating Diverse Data Sources
Data integration involves combining data from different sources to provide a unified view. Effective integration techniques enhance the overall business intelligence framework. Common methods include:
- ETL (Extract, Transform, Load): This traditional approach involves extracting data from various sources, transforming it into a suitable format, and loading it into a central repository. ETL processes allow organizations to cleanse and structure data for analysis.
- Data Virtualization: This technique enables real-time integration by providing access to data across various platforms without requiring data movement. It creates a ‘virtual’ data layer, allowing users to query and analyze information seamlessly.
- API Integration: Application Programming Interfaces (APIs) facilitate the connection between different software applications, enabling them to communicate and share data dynamically. APIs are particularly useful in integrating cloud-based services.
Importance of Data Quality and Governance
Data quality is crucial for effective business intelligence. Poor-quality data can lead to incorrect insights and decisions, undermining trust in analytics. Maintaining high data quality involves rigorous processes and governance practices. Key aspects include:
- Data Cleansing: The process of identifying and correcting errors in datasets to ensure accuracy and consistency. This involves removing duplicates, correcting inaccuracies, and standardizing formats.
- Data Governance Framework: Establishing policies and procedures for data management, including data stewardship roles, data lineage tracking, and compliance protocols. A solid governance framework ensures accountability and enhances data integrity.
- Regular Audits: Conducting periodic reviews of data quality and governance practices helps identify weaknesses and areas for improvement, ensuring that data remains reliable over time.
Best Practices for Data Security and Compliance
In big data environments, security and compliance are critical to protecting sensitive information and adhering to regulatory requirements. Organizations must implement best practices to safeguard their data assets:
- Data Encryption: Encrypting data both at rest and in transit protects sensitive information from unauthorized access. This is essential in ensuring that only authorized entities can read the data.
- Access Controls: Implementing robust access control measures limits data access to authorized personnel only. Role-based access control (RBAC) ensures that individuals can only access data necessary for their roles.
- Compliance Monitoring: Regularly reviewing and updating compliance protocols ensures adherence to regulations such as GDPR or HIPAA. This involves tracking data usage and maintaining audit trails for accountability.
“Effective data integration and governance not only enhance analytical capabilities but also foster a culture of data-driven decision-making.”
Case Studies of Successful Big Data Implementation
In the digital age, the application of big data in business intelligence is not just an advantage; it’s a necessity. Companies that have embraced big data strategies have seen transformative results, impacting their operations, customer engagement, and overall profitability. This segment explores notable examples of such implementations and the outcomes achieved across various sectors, while also reflecting on the lessons learned from unsuccessful projects.
Successful Implementations in Various Sectors
Several industry leaders have successfully harnessed big data to drive innovation and efficiency. Their experiences highlight the potential of big data analytics and the transformative outcomes that can be achieved.
- Netflix: By utilizing big data to analyze viewer preferences and behaviors, Netflix has tailored its content recommendations, leading to increased user engagement and retention. The company credits data-driven decisions for a significant boost in subscriptions, reaching over 200 million users worldwide.
- Amazon: Amazon leverages big data for personalized marketing strategies, optimizing inventory management, and enhancing customer experience. Through predictive analytics, Amazon has improved its recommendation system, contributing to a reported increase in sales by 29% yearly.
- Walmart: Walmart employs big data analytics to streamline its supply chain and inventory management. By analyzing customer purchase data, the retail giant can forecast demand efficiently, reducing excess inventory and improving profit margins.
- Uber: Uber uses big data to optimize ride-sharing logistics, pricing strategies, and driver-partner performance. The application of real-time analytics has enabled Uber to reduce wait times and enhance user satisfaction significantly.
- Target: Target has successfully implemented big data to predict customer purchasing patterns and personalize marketing. An infamous example was when they identified a teenage girl’s pregnancy based on her shopping habits, demonstrating the power of data analytics in understanding consumer behavior.
Outcomes Achieved Through Big Data Initiatives
The benefits of successful big data initiatives extend beyond just revenue increases. Companies have reported improved operational efficiencies, enhanced customer satisfaction, and sustained competitive advantages as a result of their big data strategies.
- Increased Efficiency: Organizations like GE have utilized big data for predictive maintenance in manufacturing, leading to a 10-20% reduction in maintenance costs.
- Enhanced Decision-Making: Companies such as Procter & Gamble leverage big data insights for product development and marketing strategies, ensuring their decisions are informed by actual consumer data.
- Improved Customer Engagement: The banking sector, particularly JPMorgan Chase, has implemented big data analytics to better understand customer needs, enabling them to offer personalized financial products and services.
Lessons Learned from Failed Big Data Projects
While many companies have achieved significant success with big data, several have faced challenges that resulted in project failures. Understanding these pitfalls can help organizations avoid similar mistakes.
- Lack of Clear Objectives: Many companies initiate big data projects without a clear business goal. For example, a retail chain invested significantly in big data tools without defining how it would address specific sales challenges, resulting in wasted resources.
- Poor Data Quality: Organizations that do not prioritize data quality often face challenges in achieving reliable results. A telecommunications company struggled with inconsistent data across departments, leading to unreliable insights and poor strategic decisions.
- Insufficient Skill Sets: Companies frequently underestimate the need for skilled data professionals. Organizations that fail to invest in training or hiring data scientists often find themselves unable to interpret big data effectively, leading to uninformed conclusions.
- Overreliance on Technology: Some businesses expect technology alone to drive success without integrating human insights. A well-known healthcare provider faced backlash when its data analytics team relied solely on algorithms, neglecting the importance of clinician insights in patient care analytics.
Understanding the lessons from failed data initiatives is as crucial as celebrating successes; it provides a roadmap for future endeavors.
Future Trends in Big Data and Business Intelligence
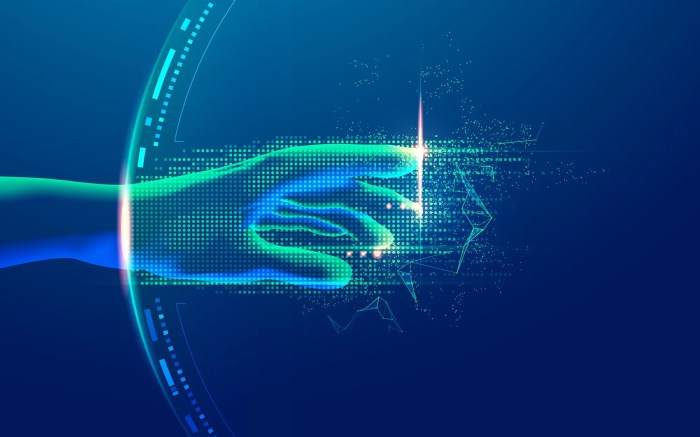
The landscape of big data and business intelligence is rapidly evolving, propelled by advancements in technology and the shifting needs of businesses. Organizations are increasingly recognizing the potential of big data to drive decision-making and foster innovation. This section explores the emerging trends that are shaping the future of big data in business intelligence, the challenges that may arise as these technologies evolve, and the significant impact of artificial intelligence (AI) and machine learning (ML) on big data analytics.
Emerging Trends in Big Data and Business Intelligence
Several key trends are currently influencing how businesses utilize big data in their decision-making processes:
- Real-time Analytics: The demand for real-time data processing is growing, enabling businesses to make immediate, informed decisions. Companies are increasingly investing in technologies that allow for continuous data integration and analysis.
- Data Democratization: Organizations are moving towards empowering all employees, not just data specialists, to access and analyze data. This trend fosters a culture of data-driven decision-making across all levels of a business.
- Cloud-based Solutions: The adoption of cloud technologies facilitates scalable data storage and processing capabilities, allowing businesses to leverage big data without significant infrastructure investments.
- Edge Computing: By processing data closer to the source, edge computing reduces latency and enhances real-time data analytics, which is crucial for applications in IoT and mobile technologies.
- Enhanced Data Privacy and Security: As data breaches become more prevalent, companies are focusing on implementing robust security measures and compliance protocols to protect sensitive information.
Challenges in Evolving Big Data Technologies
As big data technologies continue to evolve, organizations may face several challenges that could hinder their progress:
- Data Overload: The sheer volume of data generated can overwhelm organizations, making it challenging to extract valuable insights without the right tools and strategies in place.
- Integration Complexities: Merging data from multiple sources can be complicated and may require substantial resources and expertise, leading to potential inaccuracies in analysis.
- Skill Shortage: The demand for data scientists and analysts continues to outpace supply, leaving many organizations struggling to find qualified personnel to drive their big data initiatives.
- Compliance Issues: Navigating the landscape of data privacy regulations, such as GDPR and CCPA, can pose significant challenges for businesses, requiring them to adapt their data handling practices.
Impact of Artificial Intelligence and Machine Learning on Big Data Analytics
Artificial intelligence and machine learning are transforming how organizations analyze and derive insights from big data. Their integration into business intelligence processes facilitates more sophisticated analytical capabilities:
- Automated Insights: AI algorithms can process massive datasets rapidly to uncover hidden patterns and trends, delivering insights that might be difficult to identify through traditional methods.
- Predictive Analytics: Machine learning models can forecast future trends based on historical data, enabling businesses to make proactive decisions and optimize their strategies.
- Personalization: AI-driven analytics allow for the customization of products and services to meet individual customer preferences, enhancing customer satisfaction and loyalty.
- Natural Language Processing (NLP): AI technologies can analyze unstructured data, such as social media content and customer feedback, to provide deeper insights into customer sentiment and market trends.