Impact of big data on customer experience is transforming the landscape of businesses as we know it, reshaping how companies interact with their customers. As industries generate vast amounts of data, the insights gleaned from this information are becoming crucial for enhancing customer engagement and satisfaction. From personalized marketing to real-time support, big data is not just a buzzword; it’s a pivotal force driving change in customer dynamics.
Delving into the fundamental concepts of big data, we uncover how various technologies analyze extensive datasets, offering companies the ability to understand customer behavior and preferences on a granular level. This newfound knowledge enables businesses to segment their audiences effectively, tailor their services, and predict future trends, ultimately leading to a more personalized and satisfying customer experience.
Understanding Big Data Analytics
Big data analytics is a critical area in contemporary business, enabling organizations to gain valuable insights from large and complex datasets. In an era where data is generated at unprecedented rates, understanding the fundamental concepts and components of big data is essential for enhancing customer experience and driving strategic decisions.Big data refers to the enormous volumes of structured and unstructured data generated from a variety of sources.
Its components can be categorized into three main characteristics known as the “Three Vs”: Volume, Velocity, and Variety. Volume refers to the sheer amount of data generated, which can range from terabytes to petabytes. Velocity signifies the speed at which data is created and processed, often in real-time. Variety encompasses the different formats of data, including text, images, videos, and sensor data.
Types of Data Generated Across Industries
Different industries produce diverse types of data, which can be classified into several categories. Recognizing these types is vital for effective big data analytics. Here are some common data types generated across various sectors:
- Transactional Data: This type is prevalent in sectors like retail and finance, comprising details of sales transactions, payment records, and customer purchase behavior.
- Social Media Data: Platforms like Facebook and Twitter generate extensive user-generated content, including posts, comments, likes, and shares, which can be analyzed for sentiment and trends.
- Machine Data: Generated by the Internet of Things (IoT) devices and sensors, this data includes logs, metrics, and telemetry data from machinery, often used in manufacturing and logistics.
- Web Data: Analytics from website traffic, including user behavior, click patterns, and engagement metrics, are critical for businesses aiming to enhance user experience.
- Geospatial Data: Location-based data generated by GPS and mapping services aids in understanding geographical trends and optimizing logistics in various industries.
Understanding these data types helps organizations tailor their analytics strategies to meet specific business needs.
Technologies Used for Big Data Analytics
The effectiveness of big data analytics is heavily reliant on the technologies deployed. A variety of tools and platforms have emerged to handle the complexities associated with big data. Some of the key technologies include:
- Apache Hadoop: An open-source framework that allows for distributed processing of large datasets across clusters of computers, making it easier to store and analyze massive amounts of data.
- Apache Spark: A fast and general-purpose cluster computing system that provides an interface for programming entire clusters with implicit data parallelism and fault tolerance.
- NoSQL Databases: Non-relational databases like MongoDB and Cassandra are designed to handle unstructured data efficiently, providing flexibility in data storage and retrieval.
- Data Warehouse Solutions: Platforms such as Amazon Redshift and Google BigQuery enable organizations to store and analyze structured data from diverse sources for better decision-making.
- Machine Learning Algorithms: Tools like TensorFlow and Scikit-learn empower businesses to leverage predictive analytics by enabling machines to learn from data patterns and make informed predictions.
These technologies collectively enhance the capability of organizations to extract meaningful insights from big data, thereby improving customer experience and operational efficiency.
“Big data is not about the data itself, but about the insights that can be drawn from it.”
In today’s data-driven world, understanding how big data impacts decision making is essential for businesses to thrive. With vast amounts of information available, organizations can leverage insights to make informed choices that enhance operational efficiency. For a deeper dive into this transformative effect, check out the article on how big data impacts decision making , which explores the nuances of data analysis in shaping business strategies.
The Role of Big Data in Customer Insights: Impact Of Big Data On Customer Experience
Big data has profoundly reshaped how businesses understand and engage with their customers. By harnessing vast amounts of data generated from various sources, companies can gain deeper insights into customer behavior, preferences, and needs. This transformation in customer profiling allows for more personalized experiences and targeted marketing strategies.Big data facilitates a more nuanced approach to customer insights by enabling businesses to analyze patterns and trends derived from multiple data points.
Companies leverage this capability to refine customer segmentation and improve their marketing efforts. Through continuous analysis, businesses can identify distinct customer groups and tailor their offerings accordingly.
Transformations in Customer Profiling
The evolution of customer profiling through big data has led to more accurate and dynamic representations of consumers. Traditional profiling techniques often relied on limited demographic information; however, with big data, companies can analyze a multitude of factors, including online behavior, purchase history, and social media interactions. This wealth of information allows for the creation of more detailed and actionable customer profiles.For instance, a retail brand might utilize big data to segment its customer base not just by age or location, but also by purchasing patterns, preferred channels of communication, and even interests inferred from social media activity.
This multidimensional profiling helps businesses refine their marketing strategies, ensuring that messages resonate with the intended audience.
Examples of Enhanced Customer Segmentation
Companies across various industries employ big data to enhance customer segmentation, leading to more effective marketing strategies. Notable examples include:
Amazon
Ensuring data integrity in relational databases is crucial for maintaining accurate information. One of the best ways to achieve this is by implementing constraints and validations within the database schema, as detailed in the article on Best ways to ensure data integrity in relational databases. These strategies help in minimizing errors and ensuring that the data adheres to predefined rules.
The e-commerce giant uses big data analytics to segment its customers based on browsing history, purchase behavior, and wishlist items. This allows Amazon to offer personalized recommendations and targeted advertisements, significantly increasing conversion rates.
Netflix
By analyzing viewing habits and preferences, Netflix segments its audience into various categories, enabling the platform to recommend content tailored to individual users. This not only improves user experience but also enhances customer retention.
Coca-Cola
Through the analysis of social media interactions and purchase data, Coca-Cola identifies emerging trends and customer preferences, allowing the company to develop targeted marketing campaigns that resonate with specific demographics.
Leveraging Data to Predict Customer Behavior
Predictive analytics powered by big data plays a crucial role in forecasting customer behavior. Businesses can utilize various methods to analyze historical data and identify patterns that predict future actions. This proactive approach allows companies to tailor their strategies effectively.The following methods are commonly used to predict customer behavior:
1. Churn Prediction Models
Businesses analyze past customer interactions and behaviors to identify signals indicating a likelihood of churn. For example, a telecom company might notice a pattern where customers who frequently call customer service are more likely to leave, prompting targeted retention strategies.
2. Purchase Pattern Analysis
Retailers can track and analyze purchasing trends to anticipate future buying behaviors. An example includes grocery stores utilizing loyalty card data to predict which products will be in demand based on seasonal trends or past purchase behavior.
3. Sentiment Analysis
By analyzing customer reviews and social media mentions, companies can gauge customer sentiment and predict potential shifts in behavior. For example, if a significant number of customers express dissatisfaction with a product, a company might anticipate a drop in sales and adjust its marketing approach accordingly.
4. A/B Testing
This method allows companies to test different marketing strategies on segments of their audience, providing insights into which approach drives better engagement or conversions. For example, an online retailer might test two different promotional emails to see which design leads to more clicks and purchases.Through these methodologies, businesses harness the power of big data to not only understand their customers better but also to anticipate their needs and behaviors, leading to improved customer experiences and enhanced loyalty.
Enhancing Customer Experience through Personalization
The convergence of big data and customer experience has paved the way for unprecedented levels of personalization in marketing. With the insights derived from vast amounts of data, businesses are now equipped to tailor their interactions and offerings to meet individual customer preferences. This transformation not only fosters a deeper connection with consumers but also drives brand loyalty and increased sales.Big data enables personalized marketing strategies by analyzing extensive datasets that encompass customer behaviors, preferences, and interactions.
By leveraging advanced analytics, businesses can segment their audience more effectively, allowing for targeted campaigns that resonate with specific customer needs. This leads to a more engaged consumer base, as marketing efforts are no longer one-size-fits-all but rather finely tuned to individual tastes and interests.
Creating Customized Customer Interactions Using Data
The process of creating customized customer interactions involves several key steps, each of which plays a critical role in enhancing the overall customer experience. These steps include gathering data, analyzing customer behavior, segmenting the audience, and crafting personalized messages.Collecting data from various touchpoints such as social media, website interactions, and purchase history provides a comprehensive view of customer preferences. This data is then processed using analytics tools to uncover patterns and insights that inform marketing strategies.
Once the data is analyzed, businesses can segment their audience based on shared characteristics, behaviors, or preferences, enabling targeted marketing efforts. The final step involves tailoring communication and offers to meet the specific desires of each segment, resulting in a more personalized experience for customers.Examples of successful personalized campaigns driven by big data highlight the effectiveness of this approach. Notable instances include:
- Amazon: The e-commerce giant utilizes big data analytics to recommend products based on past purchases and browsing history, driving significant sales increases.
- Netflix: By analyzing viewing habits, Netflix personalizes content recommendations, improving user engagement and satisfaction.
- Coca-Cola: The “Share a Coke” campaign was tailored by using data on consumer preferences to print individual names on bottles, creating a personal connection with customers.
- Spotify: Personalized playlists such as “Discover Weekly” are generated using advanced algorithms that analyze listening habits, enhancing user experience and retention.
These examples illustrate how big data facilitates not only the personalization of marketing efforts but also the cultivation of lasting relationships between brands and their consumers, ultimately transforming how businesses interact with their clientele.
Improving Customer Service with Real-Time Data
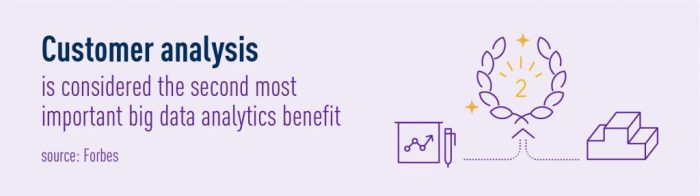
Real-time data has revolutionized customer service, enabling businesses to respond to customer inquiries and issues more efficiently than ever before. The ability to access and analyze data in real time allows companies to not only resolve customer complaints swiftly but also anticipate customer needs, significantly enhancing the overall customer experience. This immediacy fosters a responsive environment where customer satisfaction can flourish, establishing a solid foundation for loyalty and retention.The impact of real-time data on customer service efficiency is profound, as it allows organizations to streamline their operations and enhance the quality of interactions.
With technologies that facilitate immediate customer support, businesses can manage queries and issues as they arise, leading to a more proactive approach. Real-time data analytics empowers service representatives with insights that allow them to address customer needs swiftly, thus reducing wait times and increasing resolution rates.
Technologies Facilitating Immediate Customer Support
The integration of various technologies has made real-time customer support not only possible but also efficient. These tools harness the power of big data to enhance service delivery. Key technologies include:
- Chatbots: Utilizing artificial intelligence, chatbots can provide instant responses to common inquiries, guiding customers through troubleshooting processes without human intervention.
- Customer Relationship Management (CRM) Systems: These systems collect and analyze customer interactions, enabling service agents to access detailed customer histories instantly, which enhances personalized support.
- Live Chat Software: This technology allows customers to engage directly with service agents in real-time, facilitating instant communication and problem resolution.
- Social Media Monitoring Tools: By tracking customer comments and inquiries on social platforms, businesses can respond to customers in real-time, enhancing public relations and customer engagement.
To further illustrate the benefits of these technologies, consider the application of chatbots in the retail sector. Companies like Sephora have implemented AI-driven chatbots that handle a significant percentage of customer inquiries, allowing human agents to focus on more complex issues. This not only improves response times but also leads to higher customer satisfaction rates.
Methods for Analyzing Customer Feedback Quickly
Analyzing customer feedback in real-time is crucial for businesses aiming to refine their services and enhance customer satisfaction. Leveraging big data analytics enables organizations to process feedback rapidly and implement necessary changes. The following methods showcase effective strategies for quick analysis of customer feedback:
- Sentiment Analysis: By using natural language processing, businesses can analyze customer sentiments expressed in reviews and social media comments, allowing for swift adjustments based on public perceptions.
- Text Analytics: This method helps in extracting meaningful patterns from unstructured feedback data, categorizing responses for easier interpretation and quicker action.
- Real-Time Dashboarding: Implementing dashboards that aggregate customer feedback data allows for immediate visibility into customer satisfaction scores and emerging trends, facilitating quick decision-making.
- Feedback Loop Integration: Creating a system where customer feedback is continuously fed back into service processes ensures that insights are acted upon in real-time, enhancing service delivery.
For example, companies like Amazon utilize advanced text analytics to monitor customer reviews and feedback continuously. By employing these methods, they can swiftly identify areas for improvement, thus maintaining a competitive edge in customer service excellence.
Challenges and Ethical Considerations in Big Data Usage
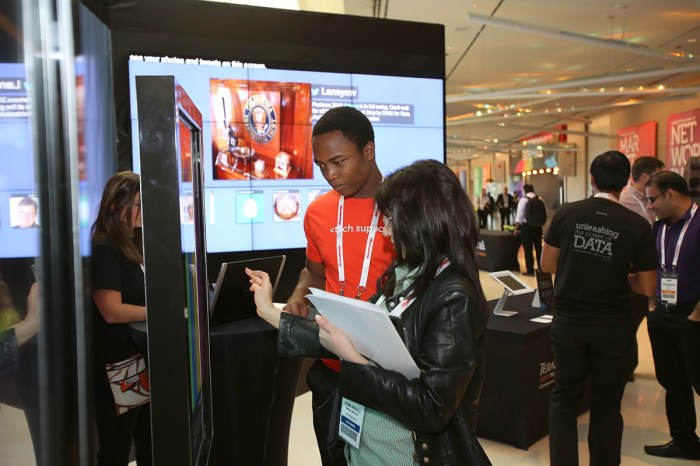
The integration of big data into customer experience strategies has rapidly transformed how businesses operate, offering enhanced insights and personalized interactions. However, this transformation comes with significant challenges and ethical considerations that organizations must navigate to protect both their customers and their reputations. Understanding these complexities is essential for leveraging big data responsibly and effectively.
Potential Risks Associated with Big Data in Customer Experience, Impact of big data on customer experience
While big data can enhance customer interactions, it also poses several risks that can impact both customers and businesses. Organizations must be aware of these challenges to mitigate potential negative outcomes. Key risks include:
- Data Breaches: The increasing volume of collected data makes systems more vulnerable to cyberattacks. A breach can lead to unauthorized access to sensitive customer information, leading to financial loss and reputational damage.
- Data Overload: With an abundance of data, organizations can struggle to extract meaningful insights. This data overload can result in poor decision-making and hinder effective customer engagement strategies.
- Misinterpretation of Data: Inaccurate data analysis can lead to misguided business strategies that do not align with customer needs, ultimately harming customer satisfaction and loyalty.
Importance of Data Privacy and Security in Handling Customer Data
Data privacy and security are critical components of managing customer data, especially in an era where consumers are increasingly aware of their rights. Organizations must prioritize the protection of personal information to maintain trust and comply with regulations. Key aspects include:
- Compliance with Regulations: Adhering to laws such as the General Data Protection Regulation (GDPR) ensures that customer data is handled legally and ethically. Non-compliance can lead to severe penalties.
- Transparency with Customers: Being open about data collection practices and how customer information is used fosters trust and encourages customers to share their data willingly.
- Implementation of Robust Security Measures: Utilizing encryption, secure access controls, and regular security audits helps safeguard customer data against potential breaches.
Ethical Implications of Using Big Data for Customer Analytics
The deployment of big data analytics raises several ethical concerns that organizations must address to uphold their integrity and customer trust. Ethical considerations include:
- Informed Consent: Customers should be fully informed about how their data will be used and have the option to opt-out. This respects their autonomy and builds trust.
- Bias in Data Analysis: Algorithms used in big data analytics can perpetuate existing biases if not carefully monitored. Ensuring fairness in analytics is essential to avoid discriminatory practices.
- Impact on Vulnerable Populations: Organizations must consider the potential for big data to exploit vulnerable groups through targeted marketing strategies that may mislead or disadvantage them.
“The responsible use of big data not only enhances customer experiences but also upholds ethical standards and protects individual rights.”