Importance of big data in financial services cannot be overstated; it serves as the backbone for innovative strategies that revolutionize the industry. In an era defined by rapid technological advancements, financial institutions are increasingly leveraging big data to enhance risk management, personalize customer experiences, and detect fraud in real-time. This transformation not only helps in meeting regulatory compliance but also paves the way for future trends that will redefine how finance operates.
Through sophisticated data analysis methodologies, financial firms can assess risks more accurately, tailor services to individual client preferences, and employ advanced tools for fraud detection. With real-world examples showcasing these applications, the financial sector stands at the forefront of a technological revolution driven by big data.
The Role of Big Data in Risk Management
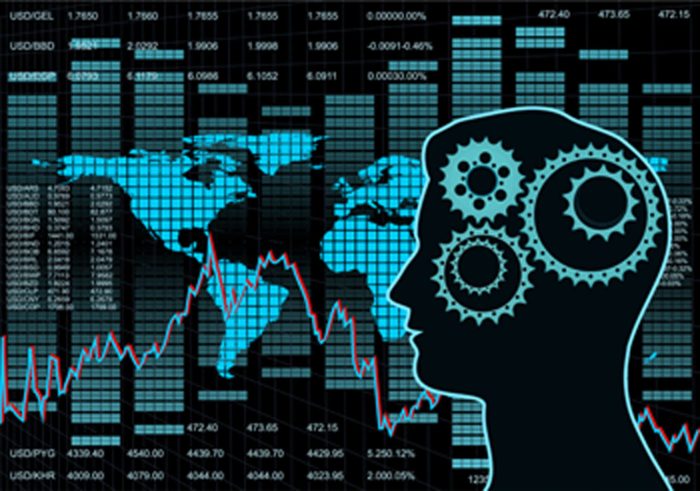
In the fast-paced financial services industry, effective risk management is crucial for maintaining stability and protecting assets. Big data plays a transformative role in enhancing risk assessment, enabling financial institutions to navigate uncertainties with greater precision. By harnessing vast amounts of data from diverse sources, organizations can identify, analyze, and mitigate potential risks more effectively than ever before.Big data contributes significantly to risk assessment by providing a comprehensive view of financial activities, customer behaviors, and market trends.
Financial institutions leverage advanced analytics and machine learning algorithms to sift through large datasets, uncovering patterns that may indicate emerging risks. This proactive approach allows institutions to assess credit risk, market risk, operational risk, and liquidity risk with a level of detail and accuracy that was previously unattainable.
Methodologies for Identifying Potential Risks
Various methodologies are employed by financial institutions to identify potential risks through data analysis. These methodologies rely on statistical models, predictive analytics, and real-time data monitoring to deliver insights into risk exposure. Key methodologies include:
- Predictive Modeling: This technique uses historical data to forecast future risks. By analyzing past behaviors and outcomes, financial institutions can create models that predict the likelihood of defaults or market downturns.
- Stress Testing: Financial institutions simulate extreme market conditions to evaluate the resilience of their portfolios. This involves analyzing how different scenarios—from economic downturns to sudden market shocks—could impact their financial stability.
- Value at Risk (VaR): VaR is a widely used risk measurement technique that estimates the potential loss in value of an asset or portfolio over a defined period for a given confidence interval. It helps institutions gauge their exposure and make informed decisions to manage risk.
A notable example of a financial institution successfully implementing big data for risk management is JPMorgan Chase. The bank utilizes a vast array of data sources, including transaction data and social media insights, to develop a more nuanced understanding of credit risk. By integrating big data analytics into its risk management framework, JPMorgan can predict potential loan defaults with improved accuracy, thereby minimizing losses and enhancing overall portfolio performance.Another example is American Express, which employs machine learning algorithms to analyze consumer spending patterns.
By identifying unusual spending behaviors in real-time, American Express can promptly detect potential fraud, thus protecting both the company and its customers from significant financial losses.In conclusion, big data serves as a cornerstone for robust risk management in financial services, empowering institutions to proactively identify and combat potential risks. By leveraging advanced methodologies and analytics, these organizations can navigate the complexities of the financial landscape with greater assurance.
Enhancing Customer Experience with Big Data
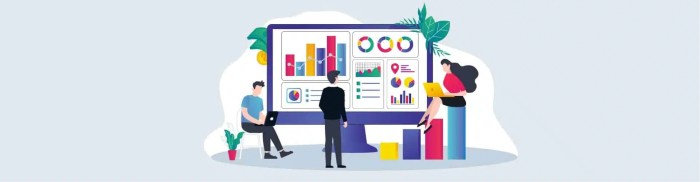
In today’s competitive financial services industry, leveraging big data is essential for enhancing customer experience. Financial institutions can harness vast amounts of data to gain insights into customer behaviors, preferences, and needs, enabling them to deliver personalized services that significantly improve customer satisfaction.By utilizing big data analytics, financial services can implement strategies that tailor customer interactions and create more meaningful relationships.
These strategies include segmentation based on behavior analysis, personalized marketing campaigns, and real-time customer service improvements. The focus is not solely on acquiring new customers but also on enhancing the experience of existing customers to foster loyalty and retention.
Strategies for Personalizing Customer Interactions
To effectively personalize customer interactions, financial services can adopt the following strategies:
- Customer Segmentation: By analyzing transaction history, demographics, and behavior patterns, financial institutions can segment their customer base. For instance, a bank may identify a group of tech-savvy millennials who prefer mobile banking and tailor specific services, such as instant loan approvals, to meet their needs.
- Predictive Analytics: Utilizing predictive analytics allows financial services to anticipate customer needs before they arise. For example, if data indicates that a customer is nearing retirement, the bank can proactively offer tailored investment advice and retirement planning services.
- Personalized Communication: Big data enables institutions to communicate with customers on platforms they prefer, at times that work best for them. This can include personalized emails or targeted ads based on their latest interactions with the institution.
- Real-time Customer Service: By using big data tools, financial institutions can enhance their customer service by providing real-time support. For example, chatbots powered by data analytics can handle inquiries based on previous customer interactions, improving response times and satisfaction.
- Feedback Loops: Collecting and analyzing customer feedback through surveys and social media allows financial services to adjust their offerings swiftly. An institution could discover through feedback that a particular service is lacking, leading to immediate improvements.
Case Studies Showcasing Improved Customer Satisfaction
Several financial institutions have successfully implemented big data strategies to enhance customer satisfaction:
- Capital One: By utilizing big data analytics, Capital One tailored credit card offers to customers based on their spending behaviors. This approach not only increased acceptance rates but also improved customer satisfaction, with many customers expressing appreciation for the relevance of the offers they received.
- American Express: American Express employs big data to enhance its fraud detection systems. By analyzing transaction patterns in real-time, the company can promptly warn customers of suspicious activities, significantly improving their security perception and overall experience.
- Bank of America: Through its mobile app, Bank of America uses big data analytics to provide personalized financial insights and budgeting tools to users. This proactive approach has led to higher user engagement and satisfaction scores.
Comparison of Traditional Customer Service Methods versus Data-Enhanced Approaches
The shift from traditional to data-enhanced customer service methods has been transformative in the financial services sector. The following comparison highlights key differences:
Aspect | Traditional Customer Service | Data-Enhanced Customer Service |
---|---|---|
Response Time | Typically slower, often requiring manual input and longer wait times. | Real-time responses driven by data analytics, leading to immediate resolutions. |
Personalization | Generic service offerings with limited personalization based on customer profiles. | Highly personalized services and recommendations based on detailed customer insights. |
Proactivity | Reactive support, addressing issues as they arise. | Proactive engagement through predictive analytics, anticipating customer needs. |
Feedback Utilization | Customer feedback is collected infrequently and often goes unaddressed. | Continuous collection and analysis of feedback, leading to ongoing service improvements. |
The integration of big data into customer service strategies not only enhances customer experience but also builds long-term loyalty, essential for the sustained growth of financial institutions. By embracing these approaches, financial services can significantly differentiate themselves in a crowded marketplace.
Fraud Detection and Prevention: Importance Of Big Data In Financial Services
Big data analytics is revolutionizing the financial services industry, particularly in the realm of fraud detection and prevention. With the rise of digital transactions and an increase in sophisticated fraudulent schemes, financial institutions are leveraging vast datasets to identify and mitigate fraudulent activities in real-time. This capability not only enhances security but also fosters trust among customers, ensuring the integrity of financial systems.The implementation of big data analytics in fraud detection involves the use of advanced algorithms and machine learning techniques that analyze transaction patterns and user behaviors.
By monitoring vast amounts of data from various sources—such as transaction histories, social media, and device fingerprints—financial institutions can identify anomalies that may indicate fraudulent activities. This proactive approach allows for immediate intervention and reduces the potential losses associated with fraud.
Technological Tools and Frameworks for Fraud Prevention
To effectively combat fraud, various technological tools and frameworks have been developed that utilize big data analytics. These tools enhance the detection capabilities of financial institutions and provide a robust defense against potential threats. Some of the key technologies include:
Machine Learning Algorithms
Algorithms such as decision trees, neural networks, and clustering techniques are employed to classify transactions as legitimate or suspicious based on historical data patterns.
While big data in healthcare offers significant benefits, it also presents substantial hurdles. Understanding what are the challenges of big data analytics is crucial for organizations aiming to leverage data effectively. Issues like data quality, integration, and the need for skilled personnel can impede progress. Therefore, addressing these challenges is essential for maximizing the potential of big data initiatives within the healthcare sector.
Real-Time Analytics Platforms
Solutions like Apache Kafka and Apache Flink enable the processing of data streams in real time, allowing for immediate response to potential fraud.
Behavioral Analytics
Monitoring user behavior through advanced analytics helps in identifying deviations from normal activity, which can be indicative of fraudulent behavior.
Predictive Modeling Tools
In the rapidly evolving healthcare industry, the integration of big data applications in healthcare industry has become indispensable. From predictive analytics to personalized medicine, big data enables healthcare providers to enhance patient outcomes and streamline operations. However, as organizations harness these capabilities, they must also navigate the complexities of data management and privacy concerns, which leads to discussions about what are the challenges of big data analytics.
Tools that utilize historical data to forecast potential fraud scenarios, providing insights that guide preventative measures.
Data Visualization Software
Platforms like Tableau or Power BI enable analysts to visually interpret data patterns, making it easier to identify trends associated with fraud.The following table Artikels the differences between traditional and big data approaches to fraud detection:
Aspect | Traditional Approach | Big Data Approach |
---|---|---|
Data Volume | Limited datasets, primarily focused on historical records. | Handles vast datasets from multiple sources, including real-time data. |
Detection Speed | Slower detection rates, often reactive rather than proactive. | Real-time detection capabilities with immediate alerts for suspicious activities. |
Analytical Techniques | Relies on rule-based systems and manual reviews. | Utilizes advanced machine learning and AI algorithms for analysis. |
Scalability | Limited scalability due to manual processes. | Highly scalable, can adapt to increasing data volumes effortlessly. |
Accuracy | Higher false positive rates due to rigid rules. | Reduced false positives and increased accuracy through continuous learning. |
The integration of big data analytics in fraud detection not only enhances security but also streamlines operations for financial institutions. The ongoing evolution of these technologies will continue to play a pivotal role in safeguarding financial transactions against the ever-evolving landscape of fraud.
Regulatory Compliance and Big Data
Big data plays a crucial role in helping financial institutions navigate the complex landscape of regulatory compliance. With an ever-evolving set of regulations, institutions must leverage data analytics to ensure adherence to local and international laws. This not only protects the integrity of the financial system but also builds trust with consumers and regulators alike.The integration of big data into compliance strategies provides financial institutions with the tools needed to monitor transactions, identify potential compliance issues, and implement corrective actions swiftly.
By harnessing vast amounts of data, organizations can uncover patterns and anomalies that may indicate non-compliance or risk exposure. Effective utilization of big data facilitates proactive compliance measures, which are essential in today’s dynamic regulatory environment.
Best Practices for Utilizing Big Data in Compliance
To effectively meet compliance requirements, financial institutions should adopt several best practices when implementing big data solutions. These approaches not only enhance compliance efforts but also improve overall operational efficiency.
- Data Governance: Establishing a robust data governance framework ensures that data quality, integrity, and security are maintained. This involves defining data ownership, access rights, and retention policies to comply with regulatory standards.
- Real-time Analytics: Utilizing real-time data analytics enables institutions to monitor transactions as they occur, allowing for immediate identification of suspicious activities that could indicate compliance breaches.
- Machine Learning Models: Implementing machine learning algorithms can help in predicting potential compliance issues by analyzing historical data and identifying trends, thereby allowing institutions to mitigate risks before they escalate.
- Regular Audits and Reporting: Conducting regular audits and maintaining thorough documentation of compliance processes and findings is essential for meeting regulatory expectations and demonstrating accountability.
“The challenge for organizations lies in balancing the need for data innovation with the strictures of regulatory compliance. A robust big data strategy can turn compliance from a burden into a competitive advantage.”
Integrating big data into compliance efforts is not without its challenges. Organizations must navigate issues such as data silos, the complexity of integrating disparate data sources, and the need for skilled personnel to analyze and interpret large datasets. However, by following best practices and leveraging advanced analytics, financial institutions can create a strong compliance culture that not only meets regulatory demands but also enhances their overall business objectives.
Future Trends of Big Data in Financial Services
The financial services sector is on the verge of a transformation driven by big data analytics. As technologies evolve and customer expectations rise, the integration of big data into financial operations is set to deepen, yielding insights that can significantly enhance decision-making processes. Understanding these future trends is essential for financial institutions aiming to remain competitive and responsive to market changes.Advancements in technology are poised to enhance big data capabilities, allowing financial service providers to make data-driven decisions with greater accuracy and speed.
Key trends indicate an increasing reliance on advanced analytics, machine learning, and artificial intelligence to process vast datasets, leading to innovative solutions that can redefine customer engagement and risk management.
Emerging Trends in Big Data Analytics, Importance of big data in financial services
Several emerging trends are shaping the future landscape of big data within financial services. These trends reflect the growing complexity and volume of data being generated in the industry.
- Rise of Predictive Analytics: Financial institutions are leveraging predictive analytics models to forecast market trends and customer behavior. By analyzing historical data, organizations can make informed predictions which enhance operational efficiency and strategic planning.
- Real-time Data Processing: With the advent of streaming data technologies, financial firms can now analyze data in real-time. This capability allows for immediate insights and quicker responses to market fluctuations, thus improving competitiveness.
- Integration of IoT Data: The increasing use of Internet of Things (IoT) devices is generating massive amounts of data. Financial services are starting to incorporate this data into their analytics, providing a more comprehensive view of customer behaviors and risk factors.
- Cloud Computing for Scalability: Financial services are migrating their big data solutions to the cloud to benefit from enhanced scalability and cost-effectiveness. This transition facilitates the handling of large datasets and facilitates collaboration across geographic boundaries.
Technological Advancements Enhancing Big Data Capabilities
Technological advancements are significantly enriching the capabilities of big data analytics in financial services. These innovations are crucial for adapting to the rapidly changing financial landscape.
- Artificial Intelligence and Machine Learning: AI and ML algorithms are becoming increasingly sophisticated, enabling financial institutions to uncover insights from vast datasets with minimal human intervention. These technologies enhance the accuracy of predictions in risk assessment and customer profiles.
- Blockchain Technology: The introduction of blockchain technology enhances data security and transparency. This technology can provide unalterable records of transactions, which is vital for risk management and compliance in financial operations.
- Natural Language Processing (NLP): NLP tools are being used to analyze unstructured data sources such as customer feedback and social media sentiments. This analysis provides valuable insights into customer preferences and market sentiment.
Impact of Artificial Intelligence on Big Data Analytics
The integration of artificial intelligence into big data analytics is set to revolutionize financial services.
- Enhanced Decision Making: AI systems can analyze vast amounts of data faster than traditional methods, providing insights that allow for more informed decision-making processes.
- Risk Management Improvements: AI algorithms can identify patterns and anomalies in data that may indicate potential risks, thereby supporting proactive risk management strategies.
- Personalization of Services: AI enables financial institutions to tailor their offerings based on individual customer data, enhancing customer satisfaction and loyalty.
- Operational Efficiency: By automating routine data analysis tasks, AI frees up human resources to focus on more strategic initiatives, thereby optimizing workforce efficiency.