Key metrics for measuring business intelligence success serve as the compass guiding organizations through the intricate landscape of data-driven decision-making. In a world where information overload is commonplace, understanding which metrics to focus on can significantly enhance the effectiveness of business intelligence initiatives. The right selection not only illuminates areas for improvement but also empowers stakeholders to make informed decisions that drive growth and innovation.
This exploration delves into the essential metrics that define success in business intelligence, ranging from data quality and user engagement to timely insights and ROI. By evaluating these metrics, businesses can better navigate their strategic journeys and harness the full potential of their data assets.
Defining Business Intelligence Success Metrics
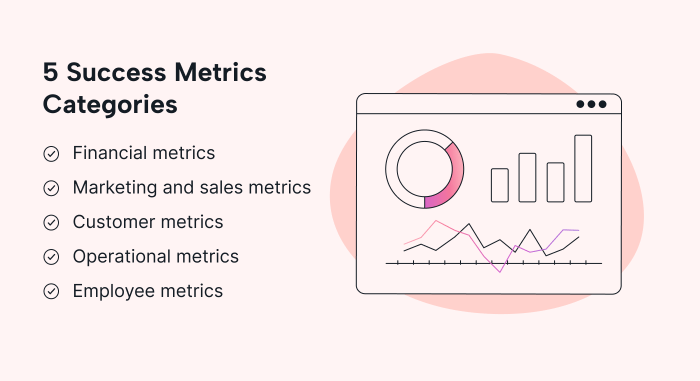
Selecting the right metrics to evaluate business intelligence (BI) initiatives is crucial for organizations aiming to leverage data for strategic decision-making. The success of BI projects often hinges on the ability to measure outcomes effectively, translating raw data into actionable insights that drive performance and growth.The importance of metrics in BI cannot be understated. They serve as the benchmarks against which organizations assess their BI efforts, providing clarity on whether initiatives meet their intended goals.
Implementing business intelligence effectively requires adherence to best practices for business intelligence implementation. These practices not only streamline processes but also enhance decision-making by ensuring that data is leveraged appropriately. Organizations that prioritize these practices can better align their strategies with data insights, leading to improved operational efficiency and competitive advantage.
Moreover, well-defined metrics facilitate communication across departments, ensuring all stakeholders have a shared understanding of success criteria.
Common Metrics for Measuring Business Intelligence Success, Key metrics for measuring business intelligence success
To effectively gauge the success of BI projects, organizations frequently utilize several common metrics. Understanding these metrics is essential for tailoring BI strategies that align with business objectives.The following metrics are often employed to measure the success of BI initiatives:
- Return on Investment (ROI): This metric assesses the financial return generated from BI investments compared to the costs incurred. A positive ROI indicates that the BI initiative contributes to the organization’s profitability.
- User Adoption Rate: The percentage of employees actively using BI tools compared to the total number of potential users. High adoption rates often correlate with effective training and tool usability.
- Data Quality Metrics: These metrics evaluate the accuracy, completeness, and reliability of the data used in BI systems. High data quality is essential for generating trustworthy insights.
- Time to Insight: This measures the time taken to convert raw data into actionable insights. Shorter times indicate efficiency in data processing and analysis.
- Data Accessibility: This metric assesses how easily users can retrieve and utilize data within BI tools. Enhanced accessibility often leads to better decision-making.
Key Performance Indicators Driving Decision-Making
Key Performance Indicators (KPIs) play a pivotal role in guiding decision-making within organizations. These indicators are derived from the metrics discussed earlier and focus on specific business objectives.KPIs are vital for establishing performance benchmarks and aligning BI initiatives with strategic goals. The following KPIs are commonly used across various industries:
- Customer Satisfaction Score (CSAT): This KPI gauges customer satisfaction levels and is crucial for understanding customer preferences and improving products or services.
- Employee Productivity: Measuring productivity helps organizations evaluate the effectiveness of BI tools in enhancing work efficiency and output.
- Sales Growth: This KPI tracks the increase in sales over a specific period, often influenced by data-driven insights from BI initiatives.
- Market Share: This metric provides insight into an organization’s performance relative to competitors, guiding strategic planning and market positioning.
- Churn Rate: This KPI reflects the percentage of customers who stop using a service over a given timeframe, informing retention strategies and customer engagement efforts.
By implementing these metrics and KPIs, organizations can better understand their BI performance, make informed decisions, and ultimately drive business success.
Data visualization plays a crucial role in business intelligence, making complex data more accessible and understandable. By highlighting the importance of data visualization in business intelligence , organizations can transform raw data into actionable insights. This clarity enables stakeholders to make informed decisions quickly, ultimately driving growth and innovation in a data-driven landscape.
Evaluating Data Quality as a Metric

In the realm of business intelligence, data quality serves as a cornerstone for effective decision-making. The accuracy and reliability of data directly influence the insights derived from analytical processes. When organizations prioritize data quality, they position themselves for enhanced operational efficiency, improved strategic planning, and greater overall success.Data quality encompasses the completeness, consistency, and accuracy of data sets, which are critical in assessing business intelligence effectiveness.
Poor data quality can lead to erroneous conclusions, misguided strategies, and ultimately, financial losses. Evaluating data quality involves several methods that help organizations gauge its impact on business intelligence outcomes.
Methods for Assessing Data Quality
Understanding and maintaining data quality requires systematic assessment methods. Here are key methods that organizations can utilize to evaluate data quality:
- Data Profiling: This process involves analyzing data from existing sources to understand its structure, content, and quality. By identifying anomalies and inconsistencies, organizations can take corrective actions.
- Data Validation: Implementing validation rules during data entry can prevent inaccuracies. This includes setting standards for data formats, ranges, and permissible values.
- Regular Audits: Conducting periodic audits of data sets helps to identify and rectify data quality issues proactively. Regular checks ensure that data remains accurate and relevant over time.
- User Feedback Mechanisms: Gathering insights from end-users can highlight data quality issues that may not be visible through automated methods. User experiences can guide necessary improvements.
The impact of data quality on decision-making processes is profound. For instance, companies relying on inaccurate sales forecasts due to faulty data may misallocate resources, leading to stock shortages or overproduction. A well-documented case is that of a retail chain that faced significant losses when its customer data was incorrect, resulting in poorly targeted marketing campaigns.
“Data quality is not just an IT issue; it is a business imperative that drives strategic performance.”
In summary, recognizing the role of data quality in business intelligence cannot be overstated. By implementing robust assessment methods, organizations can mitigate the risks associated with poor data quality, ensuring that their decision-making processes are based on reliable and accurate information.
User Adoption and Engagement Metrics

User engagement plays a pivotal role in determining the success of business intelligence (BI) tools. High user adoption rates not only validate the effectiveness of the BI solution but also ensure that the insights derived from data analytics are actively utilized in decision-making processes. Engaged users are more likely to derive value from the system, which translates into better-informed strategies and operations across the organization.The framework for measuring user satisfaction and adoption rates is essential to assess the impact of BI tools effectively.
Understanding how users interact with these systems provides insights into their preferences, challenges, and overall experience. Companies can utilize various metrics to gauge user engagement effectively, ensuring that their BI investments yield maximum returns.
Framework for Measuring User Satisfaction and Adoption Rates
To gauge user satisfaction and adoption rates, organizations can implement a structured framework consisting of several key metrics. The following points Artikel the primary components of this framework:
- Net Promoter Score (NPS): This metric assesses user loyalty by asking users how likely they are to recommend the BI tool to others. A high NPS indicates strong satisfaction and engagement.
- User Satisfaction Surveys: Regular surveys can provide qualitative insights into user experiences, challenges faced, and features appreciated. Analyzing survey results helps identify areas for improvement.
- Adoption Rate: This metric calculates the percentage of intended users actively using the BI tool. A higher adoption rate indicates better engagement and acceptance of the tool within the organization.
- Feature Utilization Rate: Tracking how frequently specific features of the BI tool are used can highlight which functionalities are most valuable to users and which may need enhancement.
- Time Spent on Platform: Monitoring the average time users spend on the BI platform can indicate engagement levels. More extended usage typically reflects greater user interest and reliance on the tool for decision-making.
The integration of these metrics provides companies with a comprehensive overview of user engagement, allowing them to address pain points and enhance the overall user experience.
Techniques for Tracking User Interactions with Business Intelligence Platforms
Organizations can adopt various techniques to monitor user interactions with BI platforms effectively. These techniques help in understanding usage patterns, identifying areas for improvement, and enhancing overall user satisfaction. The following techniques are commonly utilized:
- Clickstream Analysis: This method entails tracking users’ clicks and navigation paths within the BI tool. By analyzing this data, organizations can understand user behavior and optimize the user interface for better engagement.
- Heatmaps: Heatmaps visualize user interactions by showing where users click or hover the most. This technique helps identify popular features or areas that may require more intuitive design.
- User Session Recordings: Recording user sessions allows organizations to observe how users interact with the BI tool in real-time. This qualitative data can uncover usability issues and inform future design enhancements.
- Feedback Mechanisms: Implementing embedded feedback tools within the BI platform enables users to report issues or suggest improvements immediately. This continuous feedback loop fosters user involvement in the development process.
By employing these techniques, organizations can create a user-centric environment that enhances satisfaction and promotes higher levels of engagement with their business intelligence systems.
Time to Insight as a Performance Indicator
The speed at which organizations can generate actionable insights from their data is a critical performance indicator known as “Time to Insight.” This metric reflects how quickly businesses can convert raw data into meaningful information that drives decision-making. In a fast-paced business environment, the ability to act on insights rapidly can significantly influence overall success, impacting everything from operational efficiency to customer satisfaction.Evaluating the time it takes to turn data into insights involves a systematic approach.
This process includes several stages, from data collection to analysis and finally to implementation of insights. By measuring the time spent at each stage, organizations can identify bottlenecks and streamline their data processes.
Measuring Time to Insight
To effectively measure Time to Insight, organizations can implement a structured process consisting of the following stages:
1. Data Collection
Track how long it takes to gather raw data from various sources, including databases, application logs, and external data feeds. Efficient data collection methods can reduce this time significantly.
2. Data Preparation
Measure the time required to clean, transform, and prepare data for analysis. This stage is crucial as high-quality data leads to reliable insights.
3. Data Analysis
Record how long it takes to analyze the prepared data using analytical tools and methods. The faster the analysis, the quicker the insights emerge.
4. Insight Communication
Assess the time taken to present insights to key stakeholders. Effective communication ensures that insights are actionable and can facilitate timely decision-making.
5. Implementation
Finally, measure the time it takes for the organization to act on the insights provided. This is often where companies can see significant improvements through better processes and decision-making frameworks.By carefully tracking and optimizing these stages, businesses can significantly reduce their Time to Insight.
Impact of Faster Insights on Business Performance
Numerous organizations have demonstrated that reducing the Time to Insight can lead to substantial performance improvements. For instance, a leading retail chain implemented advanced analytics tools, allowing them to analyze customer purchasing patterns in real-time. This capability enabled them to adjust inventory levels and marketing strategies immediately, resulting in a marked increase in sales and customer satisfaction.Another example is a healthcare provider that adopted a data analytics platform to monitor patient outcomes.
By reducing the Time to Insight, the provider could quickly identify and respond to healthcare trends, leading to improved patient care and operational efficiency.These cases illustrate that faster insights not only enhance decision-making but also create a competitive advantage in rapidly changing markets. Organizations that prioritize reducing their Time to Insight are better positioned to adapt to new challenges and seize opportunities as they arise.
Return on Investment (ROI) in Business Intelligence: Key Metrics For Measuring Business Intelligence Success
Calculating the return on investment (ROI) in business intelligence (BI) is essential for organizations seeking to understand the financial impact and effectiveness of their BI tools and initiatives. By quantifying the benefits derived from BI investments, businesses can make informed decisions regarding resource allocation and strategy adjustments.To calculate the ROI of BI investments, organizations typically use the following formula:
ROI = (Net Profit from BI Investments / Cost of BI Investments) x 100
This straightforward mathematical expression allows businesses to express the value derived from their BI initiatives as a percentage. Understanding ROI goes beyond calculations; it involves considering various factors that contribute to the overall value derived from BI efforts.
Factors Contributing to the Value of Business Intelligence Initiatives
Several key factors influence the overall value that organizations derive from their business intelligence initiatives. These factors can help stakeholders appreciate the benefits of BI beyond mere financial returns. Recognizing these aspects is crucial when assessing the total impact of BI investments.
- Increased Efficiency: BI tools enable users to access data swiftly, leading to improved decision-making processes and increased operational efficiency.
- Enhanced Decision-Making: Access to real-time data and analytics empowers decision-makers to make informed choices that can positively impact the business strategy.
- Cost Reduction: By identifying inefficiencies, organizations can streamline operations and reduce costs associated with resource management.
- Revenue Growth: Insights derived from BI can uncover new market opportunities and customer segments, driving sales and increasing revenue.
- Risk Mitigation: Improved visibility into business processes allows for better risk management and the ability to anticipate potential challenges.
When presenting ROI findings to stakeholders, it is essential to adopt a structured approach that emphasizes clarity and alignment with business goals. By effectively communicating ROI, stakeholders can better understand the value and impact of BI.
Structured Approach for Presenting ROI Findings
Presenting ROI findings in a structured manner enhances stakeholder engagement and facilitates discussion around BI investments. Consider the following components when structuring your presentation:
- Executive Summary: Start with a concise overview that highlights key findings and the overall ROI percentage.
- Investment Breakdown: Provide a detailed account of the costs incurred in the BI initiative, including software, hardware, and training expenses.
- Benefits Analysis: Illustrate how the BI initiatives led to increased efficiency, revenue growth, and cost savings, supporting these claims with relevant data and case studies.
- Visual Aids: Use charts and graphs to showcase ROI trends over time and to present data comparisons that are easy to digest for stakeholders.
- Future Projections: Include forecasts that demonstrate how continued investment in BI can yield further benefits, reinforcing the strategic importance of these initiatives.
By adhering to this structured approach, organizations can convey the significance of their BI investments effectively, ensuring stakeholders grasp the critical role that business intelligence plays in driving operational success and profitability.