Role of artificial intelligence in business intelligence is increasingly becoming a pivotal aspect of modern organizations, revolutionizing how data is interpreted and decisions are made. AI technologies not only streamline data analysis but also enhance predictive capabilities, allowing businesses to foresee trends and outcomes with remarkable accuracy.
As companies integrate AI tools, they experience a transformative shift in their approach to data visualization and reporting, enabling clearer insights and more informed decision-making processes. This evolution signifies a monumental step forward in harnessing data for strategic advantage.
Importance of AI in Business Intelligence
Artificial Intelligence (AI) has transformed the landscape of Business Intelligence (BI), playing a crucial role in enhancing the decision-making processes within organizations. By leveraging vast amounts of data, AI enables businesses to derive insights and make informed choices, leading to more efficient operations and increased competitiveness. The integration of AI into BI systems not only streamlines data processing but also contributes to a deeper understanding of market dynamics and consumer behavior.
AI Enhancing Decision-Making
AI technologies provide organizations with advanced tools to analyze data at a speed and scale unattainable by human analysts. AI algorithms can identify patterns and trends within data sets that inform strategic decisions. For example, companies like Netflix utilize AI-driven recommendation systems to personalize user experiences, ultimately driving engagement and revenue. By employing AI, businesses can shift from reactive to proactive decision-making, allowing them to anticipate challenges and capitalize on opportunities more effectively.
Predictive Analytics Powered by AI, Role of artificial intelligence in business intelligence
Predictive analytics, fueled by AI, is essential in forecasting business outcomes with a high degree of accuracy. This approach involves the use of historical data to create models that predict future trends, enabling businesses to make data-driven forecasts. For instance, retail giants such as Walmart employ predictive analytics to optimize inventory levels based on projected consumer demand, significantly reducing costs and improving customer satisfaction.
Understanding business law is crucial for any entrepreneur looking to navigate the complexities of the commercial world. This comprehensive guide not only outlines the benefits of legal knowledge but also provides practical solutions that can help businesses avoid common pitfalls. By leveraging legal frameworks effectively, companies can enhance their operational efficiency and ensure long-term sustainability.
The ability to forecast sales and market trends empowers organizations to align their strategies more closely with actual market conditions.
Impact of AI on Data Visualization and Reporting
AI significantly enhances data visualization and reporting processes, transforming how insights are presented and interpreted. With the help of AI-powered data visualization tools, complex data sets can be converted into intuitive graphs and charts that make insights more accessible and actionable. For instance, platforms like Tableau utilize AI to automate data analysis and generate visual reports that highlight key performance indicators, allowing decision-makers to quickly grasp critical business metrics.
This capability not only aids in better understanding of data but also promotes transparency and collaboration across teams.
“AI not only augments the analytical capabilities of organizations but also democratizes access to insights, enabling teams at all levels to make data-informed decisions.”
To maximize success, businesses must focus on their core offerings. A useful resource for this is the Key Activities in Business Model Canvas , which delves into the essential tasks that drive value creation. By understanding these activities, entrepreneurs can streamline their processes and effectively implement strategies that enhance their business models.
AI Tools and Technologies for Business Intelligence
Artificial Intelligence (AI) has revolutionized the landscape of Business Intelligence (BI) by introducing a suite of innovative tools and technologies that enhance data analysis, visualization, and decision-making. These AI-driven solutions empower businesses to harness their data more effectively, providing insights that were previously unattainable through traditional methods. As organizations strive to remain competitive, understanding the available AI tools and their integration into existing BI frameworks becomes essential.
AI Tools Commonly Used in Business Intelligence Applications
Numerous AI tools are now integral to business intelligence applications, each offering unique functionalities that aid in data analysis and reporting. These tools facilitate the processing of large datasets, enabling businesses to derive actionable insights swiftly. The following list highlights some key AI tools utilized in the BI landscape:
- Tableau: This powerful data visualization tool incorporates AI capabilities, allowing users to analyze data and generate visual insights quickly. Its integration with AI helps automate data preparation and provides predictive analytics.
- Power BI: Microsoft’s business analytics tool leverages AI for advanced data modeling, enabling users to create interactive reports and dashboards. It uses machine learning algorithms to identify trends and outliers in datasets.
- Looker: Looker provides robust data exploration capabilities and integrates seamlessly with various databases. Its AI-powered features enable users to generate insights from data more intuitively and efficiently.
- Qlik Sense: This platform offers self-service data visualization and analytics, powered by AI to deliver actionable insights. Its associative model allows users to analyze connections in their data comprehensively.
- IBM Watson Analytics: IBM’s AI platform provides advanced analytics capabilities, allowing businesses to uncover hidden patterns and trends in their data, enriching the decision-making process.
- Google Cloud AI: This suite of machine learning tools offers businesses the ability to build AI models tailored to their specific BI needs, enhancing data processing and predictive capabilities.
Comparison of Machine Learning and Traditional Data Analysis Methods
The distinction between machine learning and traditional data analysis methods lies in their approach to data interpretation and forecasting. While traditional methods rely heavily on predefined statistical models and hypotheses, machine learning employs algorithms that can learn from data and improve over time. This section provides a succinct comparison:
Aspect | Traditional Data Analysis | Machine Learning |
---|---|---|
Data Handling | Static, predefined models | Dynamic, adapts based on new data |
Complexity | Limited to simple relationships | Can handle complex, nonlinear relationships |
Predictive Power | Fixed predictions based on established theories | Generates predictions based on patterns learned from data |
Scalability | Less scalable with increasing data | Highly scalable with large datasets |
Integration of AI with Existing Business Intelligence Platforms
Integrating AI into existing business intelligence platforms is pivotal for organizations seeking to enhance their analytical capabilities. This integration allows businesses to enrich their data analysis processes, streamline operations, and improve decision-making. Effective integration typically involves the following considerations:
- Data Compatibility: Ensuring that AI tools can seamlessly interact with existing data architectures and formats enhances the usability of business intelligence systems.
- User Training: Providing adequate training for staff on AI tools is crucial to maximize their potential and minimize resistance to new technologies.
- Scalability and Flexibility: The chosen AI solutions should be scalable and flexible enough to adapt to evolving business needs and data growth.
- Real-time Analytics: Integrating AI enables real-time data processing, allowing for immediate insights and timely decision-making.
- Cost Efficiency: Businesses should assess the cost implications of integrating AI tools with existing systems to ensure that the investment yields significant returns in efficiency and insight generation.
Use Cases of AI in Business Intelligence: Role Of Artificial Intelligence In Business Intelligence
The multifaceted role of Artificial Intelligence (AI) in business intelligence (BI) is increasingly becoming a cornerstone for organizations aiming to enhance their operational efficiency and decision-making processes. Across various industries, companies are leveraging AI technologies to transform data into actionable insights, which is vital for maintaining a competitive edge in today’s fast-paced market. This section highlights several significant use cases of AI in BI, demonstrating its impact on different sectors and the benefits it brings to businesses.
Industries Leveraging AI for Enhanced Business Insights
Numerous industries are actively utilizing AI to improve their business analytics and derive meaningful insights from vast amounts of data. The following examples illustrate how specific sectors are embracing AI technologies:
- Healthcare: AI algorithms analyze patient data to identify trends and predict health outcomes, which aids in personalized medicine and treatment plans. For instance, IBM Watson Health uses AI to assist doctors in diagnosing diseases by analyzing patient histories and medical literature.
- Finance: Financial institutions employ AI for fraud detection by evaluating transaction patterns in real-time. Companies like PayPal utilize machine learning to analyze user behavior, flagging any suspicious activity almost instantaneously.
- Retail: Retailers leverage AI for inventory management and customer insights. For example, Walmart uses AI to predict customer demand and optimize stock levels, ensuring product availability during peak shopping times.
- Manufacturing: AI in manufacturing helps predict equipment failures and optimize maintenance schedules. GE utilizes AI-driven analytics to enhance operational efficiency and reduce downtime in its manufacturing plants.
AI-Driven Customer Segmentation Improves Marketing Strategies
Customer segmentation powered by AI enables businesses to tailor their marketing strategies effectively, leading to higher engagement and conversion rates. By analyzing large datasets, AI can identify distinct customer groups based on behavior, preferences, and purchasing patterns. This segmentation allows marketers to craft personalized campaigns that resonate more deeply with each consumer segment.The significance of AI-driven customer segmentation can be illustrated as follows:
- Enhanced Targeting: AI algorithms analyze historical data to predict which segments are more likely to respond positively to specific marketing efforts.
- Dynamic Campaign Adjustments: Marketers can adjust campaigns in real-time based on AI insights, optimizing messaging and offers for different segments as market conditions change.
- Improved Customer Retention: By understanding customer needs and behaviors, businesses can develop loyalty programs that increase retention rates.
- Cost Efficiency: Targeted marketing reduces wasteful spending on broad campaigns, ensuring resources are allocated towards segments showing the most promise.
Role of AI in Real-Time Data Processing and Analysis for Businesses
The ability of AI to process and analyze data in real-time is transforming how businesses operate. This capability allows organizations to react swiftly to market changes and customer needs, resulting in improved decision-making and operational efficiency. The following points emphasize the importance of real-time data processing in business intelligence:
- Instant Insights: AI tools can analyze incoming data streams, providing businesses with immediate insights that inform strategic decisions without delay.
- Predictive Analytics: By employing AI-driven predictive models, businesses can anticipate trends and customer behaviors, allowing for proactive responses rather than reactive management.
- Enhanced Collaboration: Real-time data sharing across departments fosters a collaborative environment where teams can work together on the latest insights and insights-driven strategies.
- Adaptive Operations: AI can help businesses adapt their operations based on real-time analytics, ensuring optimal resource allocation and operational efficiency.
Challenges and Considerations in Implementing AI
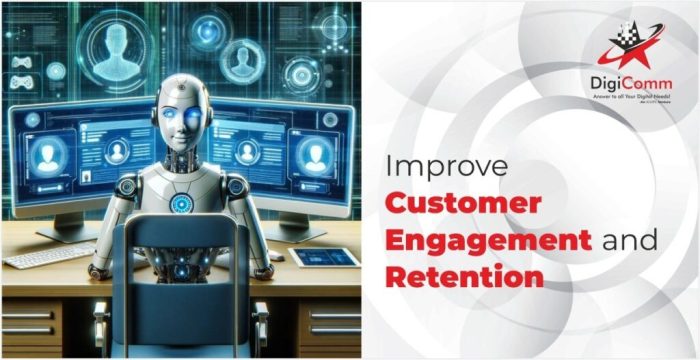
The integration of artificial intelligence (AI) into business intelligence (BI) frameworks presents organizations with significant opportunities but also numerous challenges. To harness the full potential of AI, businesses must navigate a complex landscape of technological, ethical, and operational considerations. Successful implementation requires a thorough understanding of these challenges.Implementing AI in business intelligence is not without its hurdles. Organizations face a variety of challenges, including data quality issues, the need for skilled personnel, and the complexity of integrating AI systems with existing technology.
Additionally, organizations must contend with the rapid pace of AI advancements, which can make it difficult to stay current and competitive in the market.
Potential Challenges in Integration
The challenges organizations encounter while integrating AI into their business intelligence operations can be multifaceted. Key challenges include:
- Data Quality and Quantity: AI systems rely on high-quality data to yield meaningful insights. Incomplete, biased, or inaccurate data can lead to flawed analyses and decision-making.
- Skill Gap: There is often a shortage of professionals skilled in AI technologies and data analytics. Organizations may struggle to find or train employees capable of implementing and managing AI solutions effectively.
- Integration Complexity: Merging AI systems with legacy BI tools can be technically challenging and may require significant resources to ensure compatibility and functionality.
- Change Management: Employees may resist adopting AI-driven processes, fearing job displacement or the need to adapt to new technologies. Proper change management strategies are essential for successful adoption.
- Cost of Implementation: The initial investment for AI technologies can be substantial. Organizations must evaluate the long-term benefits against the upfront costs to determine feasibility.
Ethical Considerations Related to Data Privacy
Ethical challenges surrounding data privacy and AI usage are crucial for organizations to address. As AI systems often rely on vast amounts of personal and sensitive data, there exists a heightened risk of privacy breaches and misuse of information. Businesses must ensure compliance with data protection regulations, such as the General Data Protection Regulation (GDPR), which mandates strict guidelines on data handling and user consent.
“The ethical use of AI is not only a legal obligation but also a moral responsibility for organizations.”
Organizations must establish clear policies for data governance and privacy, ensuring transparency in how data is collected, processed, and analyzed. Engaging with stakeholders and implementing robust data protection measures can help mitigate risks and build trust with customers.
Best Practices for Successful AI Implementation
Successful integration of AI into business intelligence requires adherence to best practices that can streamline the process and enhance outcomes. Organizations should consider the following best practices:
- Define Clear Objectives: Establish specific goals for AI implementation that align with broader business strategies. This will guide the selection of appropriate AI tools and technologies.
- Invest in Training: Provide ongoing education and training for staff to ensure they are equipped with the necessary skills to leverage AI effectively.
- Ensure Data Quality: Implement protocols for data management that prioritize data accuracy and integrity, which are critical for the success of AI applications.
- Foster a Collaborative Culture: Encourage collaboration between IT, data scientists, and business units to facilitate knowledge sharing and enhance the effectiveness of AI initiatives.
- Monitor and Evaluate: Continuously assess AI performance and impact on business outcomes, making adjustments as necessary to optimize results.
Future Trends of AI in Business Intelligence
The future of artificial intelligence (AI) in business intelligence (BI) is poised to transform how organizations analyze data, make decisions, and gain competitive advantages. As technology continues to advance, businesses are increasingly leveraging AI to enhance their decision-making processes, improve operational efficiencies, and drive innovation. This section explores the anticipated developments in AI technology that will shape the landscape of business intelligence in the coming years.
Predicting AI Developments Influencing Business Intelligence
The convergence of AI technologies with business intelligence is expected to yield significant advancements. Future developments will likely include greater automation of data collection and analysis, enhanced predictive analytics capabilities, and the integration of advanced machine learning algorithms. These innovations will streamline workflows and enable organizations to extract actionable insights from complex datasets with unprecedented speed and accuracy.Key trends anticipated in AI technology that may shape business intelligence include:
- Natural Language Processing (NLP): With improvements in NLP, business users will be able to interact with BI tools using conversational language. This trend will democratize data access, allowing non-technical users to query data and generate reports without needing specialized skills.
- Augmented Analytics: AI-driven augmented analytics will automate data preparation, insight generation, and sharing, making analytics more accessible and efficient. Tools will increasingly suggest insights and highlight anomalies in datasets without user intervention.
- Real-time Analytics: The implementation of AI in real-time analytics will enable organizations to make timely decisions based on the latest data. This will be crucial in fast-paced industries where market conditions can change rapidly.
- Ethical AI and Governance: As the reliance on AI grows, organizations will focus on ethical AI practices and governance frameworks to ensure responsible data use and mitigate biases in AI algorithms.
Evolving Role of AI in Decision-Making Processes
AI’s influence on decision-making within organizations continues to evolve as businesses seek to integrate advanced analytics into core operations. The ability to harness large volumes of data and derive insights will facilitate more informed strategic decisions. AI not only analyzes past trends but also predicts future outcomes, enabling organizations to adopt a proactive rather than reactive approach.Businesses will increasingly rely on AI systems to:
- Enhance Predictive Capabilities: AI models will assess various scenarios and forecast potential outcomes, leading to data-driven decision-making that minimizes risk.
- Personalize Customer Experiences: By analyzing customer behavior and preferences, AI will help businesses tailor their offerings, resulting in improved customer satisfaction and loyalty.
- Optimize Supply Chain Management: AI will streamline supply chain processes by predicting demand fluctuations and optimizing inventory management, resulting in cost savings and efficiency gains.
- Support Agile Decision-Making: AI will enable organizations to pivot quickly in response to market changes by providing real-time insights and recommendations based on current data.
Skills Needed for Workforce Adaptation to AI Advancements
As AI technologies continue to evolve, the workforce will require a new set of skills to harness the potential of AI in business intelligence effectively. Organizations must invest in training and development to ensure employees are equipped to work alongside AI systems and leverage their capabilities.Key skills that will be essential for adapting to AI advancements in business intelligence include:
- Data Literacy: Employees must possess the ability to understand and interpret data, as well as the insights derived from AI analytics.
- Technical Proficiency: Familiarity with AI tools and technologies, including machine learning algorithms and data visualization software, will be critical for effective utilization.
- Critical Thinking: The capacity to analyze AI-generated insights critically and make informed decisions will remain a vital skill in the data-driven landscape.
- Collaboration and Communication: As AI systems become integral to business processes, teamwork and the ability to communicate findings and recommendations will be essential for driving organizational success.