What is the future of big data analytics? This question sits at the crossroads of technology and innovation, beckoning us to explore a realm where massive data sets transform industries and reshape decision-making processes. As we stand on the brink of a data revolution, the integration of artificial intelligence, real-time data processing, and advanced analytics promises to usher in unprecedented opportunities and challenges.
Emerging technologies in big data analytics are set to redefine our understanding of data. From machine learning algorithms that enhance predictive analytics to the increasing importance of real-time processing for immediate insights, the landscape is evolving rapidly. Industries such as healthcare, retail, and finance are harnessing these advancements, showcasing the transformative impact of big data on decision-making and operational efficiency.
Future Trends in Big Data Analytics: What Is The Future Of Big Data Analytics
The landscape of big data analytics is rapidly evolving, driven by technological advancements and increasing data generation. As industries continue to recognize the value of leveraging data for decision-making, several key trends are emerging that promise to shape the future of analytics. The integration of artificial intelligence, the rise of real-time data processing, and the adoption of new technologies are pivotal in this transformation.
Emerging Technologies in Big Data Analytics
Several innovative technologies are gaining traction in the realm of big data analytics, enhancing the capabilities and efficiency of data processing. These technologies not only improve data analysis but also facilitate deeper insights from vast datasets. The following are notable technologies set to define the future:
- Apache Kafka: A distributed streaming platform that enables the handling of real-time data feeds, Kafka is increasingly utilized for building real-time data pipelines and streaming applications, making it pivotal for organizations seeking immediate insights.
- Edge Computing: By processing data closer to its source, edge computing reduces latency and bandwidth use, allowing organizations to analyze data in real-time, especially crucial for IoT applications.
- Data Lakes: Organizations are shifting towards data lakes for storing vast amounts of structured and unstructured data. This flexible architecture aids in accommodating diverse data formats, enabling enhanced data exploration and analytics.
- Cloud-Native Solutions: The rise of cloud computing has led to the development of cloud-native analytics solutions that offer scalability and flexibility, enabling businesses to analyze data without the constraints of on-premises infrastructure.
Role of Artificial Intelligence in Shaping Future Analytics, What is the future of big data analytics
Artificial intelligence is at the forefront of revolutionizing big data analytics, providing powerful tools for extracting valuable insights from complex datasets. AI technologies, such as machine learning and natural language processing, enhance analytical capabilities by automating processes and uncovering patterns that traditional methods might overlook. The following factors highlight the significance of AI in future analytics:
- Automated Insights: AI algorithms can analyze massive datasets quickly and efficiently, delivering actionable insights without human intervention. This capability allows organizations to make informed decisions rapidly.
- Predictive Analytics: Machine learning models can forecast trends and behaviors based on historical data, empowering businesses to anticipate market changes and adjust their strategies accordingly.
- Enhanced Decision-Making: AI-driven analytics provides deeper, data-backed insights, facilitating more strategic decision-making processes, which are essential in today’s fast-paced business environment.
- Natural Language Processing: NLP technologies enable users to query data using natural language, making analytics more accessible and allowing non-technical users to extract insights easily.
Significance of Real-Time Data Processing in Upcoming Trends
Real-time data processing is becoming increasingly crucial for organizations aiming to remain competitive. The ability to analyze and act on data as it is generated allows businesses to respond swiftly to changing market conditions and customer behaviors. The importance of real-time analytics is underscored by the following aspects:
- Instant Decision-Making: Real-time analytics equips organizations to make decisions based on current data, enabling timely responses and improving overall business agility.
- Customer Experience Enhancement: By processing data in real-time, businesses can personalize customer interactions and improve engagement, resulting in higher satisfaction and retention rates.
- Operational Efficiency: Real-time insights help streamline operations by identifying inefficiencies and bottlenecks as they occur, facilitating immediate corrective actions.
- Competitive Advantage: Organizations that harness real-time data analytics can gain an edge over competitors by being more responsive to market demands and customer needs.
Applications of Big Data in Various Industries
Big data analytics has become a cornerstone for transformation across various sectors, enabling organizations to harness vast amounts of data for informed decision-making. With advanced analytical tools, businesses are uncovering valuable insights that drive efficiency, improve customer experiences, and foster innovation. This discussion explores how big data is reshaping industries like healthcare, retail, and financial services.
Transformations in Healthcare
The healthcare sector stands as a prime example of how big data can enhance patient care and operational efficiency. By analyzing large datasets from patient records, medical imaging, and genetic information, healthcare providers can identify trends, improve diagnostics, and personalize treatment plans.
In today’s data-driven landscape, understanding how big data impacts decision making is essential for businesses aiming to stay competitive. Analyzing vast amounts of data can unveil insights that guide strategic choices and improve customer satisfaction. By leveraging big data, organizations can make informed decisions that are not only timely but also aligned with consumer trends and preferences.
- Predictive analytics enables hospitals to foresee patient influx and manage staffing accordingly, reducing wait times and enhancing service delivery.
- Big data tools aid in the development of precision medicine by analyzing genetic profiles and lifestyle data to tailor treatments.
- Wearable health devices collect real-time data, allowing for continuous monitoring of patients and timely interventions.
The integration of big data fosters a proactive approach in healthcare, shifting from reactive treatments to preventive care.
Data-Driven Decision Making in Retail
In the retail industry, big data analytics drives strategic decision-making by offering insights into consumer behavior and market trends. Retailers utilize data from various sources, including point-of-sale systems, customer loyalty programs, and online shopping patterns.
To streamline your workflows, it’s crucial to learn how to automate database maintenance tasks effectively. By implementing automation tools, you can significantly reduce downtime and ensure your database remains optimized without constant manual intervention. This not only enhances performance but also allows your team to focus on more strategic initiatives, ultimately contributing to better overall efficiency.
- Customer segmentation based on purchasing behaviors allows retailers to target marketing efforts more effectively, increasing conversion rates.
- Inventory management systems use predictive analytics to optimize stock levels, reducing excess inventory and stockouts.
- Personalized marketing campaigns, powered by data analysis, enhance customer engagement by delivering tailored recommendations and promotions.
These applications illustrate how data empowers retailers to create efficient operations and enhance customer experiences.
Impact of Big Data in Financial Services
The financial services industry leverages big data analytics to mitigate risks, enhance security, and improve customer service. By analyzing transaction data, market trends, and customer profiles, financial institutions can make informed decisions and offer better services.
- Fraud detection systems use machine learning algorithms to analyze transaction patterns and detect anomalies, safeguarding clients’ assets.
- Risk assessment models help in evaluating loan applicants by analyzing credit history and behavioral data, leading to more accurate lending decisions.
- Customer analytics allow financial firms to tailor products and services, improving customer satisfaction and retention.
The influence of big data in financial services demonstrates its capability to drive innovations and improve overall operational efficiency.
Challenges Facing Big Data Analytics
As big data analytics continues to evolve, it brings with it a host of challenges that organizations must navigate. The increasing volume, variety, and velocity of data pose not only technical obstacles but also ethical dilemmas that require careful consideration. Addressing these challenges is crucial for businesses aiming to leverage big data effectively while maintaining trust and compliance.
Ethical Concerns Surrounding Big Data Usage
The ethical concerns surrounding big data usage largely stem from issues of privacy, consent, and surveillance. Organizations often collect vast amounts of personal data without the explicit consent of individuals, leading to potential misuse and exploitation of this information.
Data ethics is paramount in ensuring that analytics do not infringe upon individual rights or contribute to discriminatory practices.
In practice, this means that businesses must develop robust frameworks and guidelines to ensure that data collection and analysis abide by ethical standards. Additionally, transparency in data usage can foster trust among consumers, thereby enhancing brand reputation and customer loyalty.
Hurdles in Data Integration from Various Sources
Integrating data from diverse sources remains one of the most significant challenges in big data analytics. Organizations often deal with structured data from databases and unstructured data from social media and IoT devices, leading to compatibility issues. A few key hurdles include:
- Data Silos: Different departments may use various systems that do not communicate effectively, preventing a holistic view of data.
- Inconsistent Formats: Data collected from multiple sources often come in different formats, complicating the integration process.
- Quality of Data: The accuracy, completeness, and timeliness of data can vary significantly, affecting the overall analytics output.
To overcome these challenges, organizations must invest in data integration tools and techniques that can standardize and harmonize data across platforms.
Importance of Data Privacy and Security Measures
As organizations increasingly rely on big data, the need for stringent data privacy and security measures becomes critical. The risks associated with data breaches can be devastating, leading to financial losses and reputational damage.
Implementing robust security frameworks protects not just the organization, but also the individuals whose data is being analyzed.
Key considerations for enhancing data privacy and security include:
- Encryption: Utilizing encryption techniques can safeguard sensitive data both at rest and in transit.
- Access Controls: Establishing strict access controls ensures that only authorized personnel have access to sensitive information.
- Regular Audits: Conducting regular audits and assessments helps identify vulnerabilities and ensure compliance with data privacy regulations.
By prioritizing data privacy and security, organizations can build a resilient analytics framework that aligns with legal requirements and ethical standards.
Skillsets Required for Future Big Data Professionals
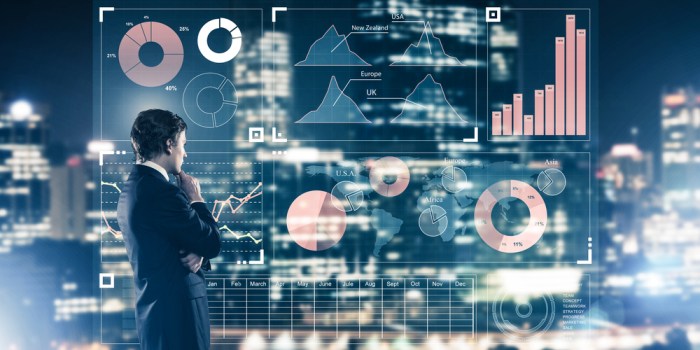
As the reliance on big data grows, so does the demand for skilled professionals who can analyze and interpret vast amounts of information. The landscape of big data requires a unique blend of technical expertise and soft skills to navigate the complexities involved in data manipulation and analysis. This segment Artikels the essential skillsets needed for individuals aspiring to excel in the big data sector.
Technical Skills for Big Data Roles
To thrive in big data roles, professionals must possess a robust set of technical skills that enable them to handle data effectively. These skills not only encompass programming and analytical abilities but also knowledge of specific tools and technologies crucial for big data analytics.
- Proficiency in Programming Languages: Knowledge of languages such as Python, R, and SQL is fundamental for data manipulation and analysis.
- Familiarity with Big Data Technologies: Understanding frameworks like Hadoop, Spark, and Kafka is essential for managing large datasets.
- Data Visualization Skills: Competence in tools such as Tableau, Power BI, or D3.js allows professionals to present complex data insights effectively.
- Machine Learning Knowledge: Familiarity with machine learning algorithms and their implementation can provide deeper insights through predictive analytics.
- Database Management: Skills in working with both structured and unstructured databases, including NoSQL databases like MongoDB and Cassandra.
Soft Skills That Enhance Success in Data Analytics Careers
While technical skills are critical, soft skills play an equally significant role in the success of data analytics professionals. These skills enhance collaboration, communication, and problem-solving capabilities, which are essential in a field that often requires teamwork and stakeholder engagement.
- Analytical Thinking: The ability to approach complex problems logically and systematically is crucial for deriving meaningful insights from data.
- Effective Communication: Being able to convey data-driven insights clearly to non-technical stakeholders is vital for influencing decision-making.
- Collaboration: Working alongside cross-functional teams fosters a better understanding of business needs and encourages innovative solutions.
- Adaptability: The fast-paced evolution of technology necessitates a willingness to learn and adapt to new tools and methodologies.
- Creativity: Creative problem-solving can lead to innovative approaches in data analysis and visualization.
Educational Pathways and Certifications Relevant to Big Data
Pursuing education and certifications tailored to big data analytics is a strategic way to build the necessary skillset for this field. Many universities and online platforms offer specialized programs designed to equip aspiring professionals with relevant knowledge.
- Degree Programs: Many universities offer bachelor’s and master’s degrees in data science, computer science, or information technology, providing a strong foundation in both theory and application.
- Online Courses: Platforms like Coursera, edX, and Udacity provide courses on big data technologies and analytics, often in partnership with leading institutions.
- Certifications: Credentials such as the Certified Analytics Professional (CAP), Cloudera Certified Associate (CCA), and Microsoft Certified: Azure Data Scientist Associate enhance professional credibility and showcase expertise.
- Bootcamps: Intensive coding bootcamps can accelerate learning and provide hands-on experience with big data tools and programming languages.
The Impact of Big Data on Decision Making
Big data analytics has revolutionized the way organizations approach decision-making. By leveraging vast amounts of data, businesses can derive actionable insights that significantly enhance their strategic choices. The integration of data-driven methodologies into business practices not only increases efficiency but also fosters innovative solutions that address real-world challenges. This transformation is particularly evident in how data visualization tools and predictive analytics shape organizational strategies.
Influence of Data Visualization Tools on Business Decisions
Data visualization tools play a critical role in translating complex datasets into accessible visual formats. These tools enable decision-makers to identify patterns, trends, and anomalies quickly, which facilitates a more intuitive understanding of data. Effective data visualization transforms raw data into meaningful insights through:
- Enhanced Clarity: Visualizations distill large datasets into charts and graphs, making it easier for stakeholders to grasp essential information at a glance.
- Improved Engagement: Engaging visuals capture attention and promote discussions among team members, leading to collaborative decision-making.
- Rapid Interpretation: When data is represented visually, decision-makers can spot trends and outliers swiftly, allowing for timely interventions.
“Data visualization allows organizations to communicate insights effectively and facilitates data-driven decision-making.”
Role of Predictive Analytics in Strategic Planning
Predictive analytics leverages statistical algorithms and machine learning techniques to forecast future events based on historical data. This capability is indispensable for organizations striving to optimize their strategic planning processes.The applications of predictive analytics in decision-making include:
- Risk Management: By identifying potential risks and forecasting their impact, businesses can develop mitigation strategies proactively.
- Market Trend Analysis: Predictive models help organizations anticipate market shifts, enabling them to adjust their strategies accordingly.
- Customer Behavior Forecasting: Understanding customer preferences through predictive insights allows companies to tailor their offerings and marketing strategies, enhancing customer satisfaction.
Comparison of Traditional Decision-Making Processes with Data-Driven Approaches
Traditional decision-making often relies on intuition and past experiences, which can lead to biases and uninformed choices. In contrast, data-driven approaches utilize quantitative analysis to guide decisions, thus minimizing subjectivity.Key differences include:
- Evidence-Based Decisions: Data-driven approaches rely on empirical evidence, reducing the influence of personal biases that may affect traditional methods.
- Adaptability: Data-driven strategies can adjust quickly to changing conditions based on real-time data, unlike traditional methods which may lag behind.
- Enhanced Accuracy: By utilizing data analytics, organizations can achieve higher precision in their decision-making processes compared to relying solely on historical experiences.
Through the integration of big data analytics, organizations can make more informed, strategic decisions that ultimately lead to enhanced performance and competitive advantage.